Power Up Qwen 3 with AMD Instinct: A Developer’s Day 0 Quickstart#
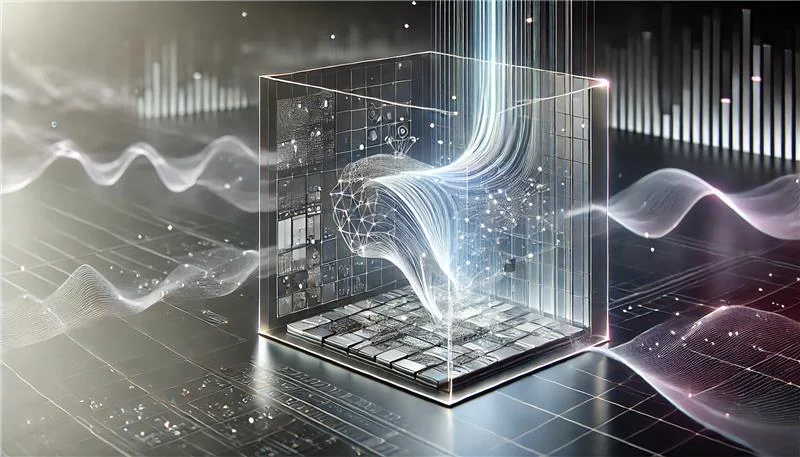
AMD is excited to announce Day 0 support for Alibaba’s latest Large Language Models Qwen3-235B Qwen3-32B Qwen3-30B on AMD Instinct™ MI300X GPU accelerators using vLLM and SGLang. In this blog we show you how to accelerate Alibaba’s cutting-edge Qwen 3 language models, featuring advanced reasoning, multilingual capabilities, and agent functionality, using AMD Instinct™ MI300X GPUs. You will learn to deploy dense and Mixture-of-Experts models with full support for vLLM and SGLang, leveraging AMD’s advanced GPU architecture for high-throughput, low-latency inference.
Brief Introduction to Qwen 3 Models#
Qwen 3 offers a comprehensive suite of dense and mixture-of-experts (MoE) models. It delivers groundbreaking advancements in reasoning, instruction-following, agent capabilities, and multilingual support, with the following key features:
Unique support of seamless switching between thinking mode (for complex logical reasoning, math, and coding) and non-thinking mode (for efficient, general-purpose dialogue) within single model, ensuring optimal performance across various scenarios.
Significant enhancement in its reasoning capabilities, surpassing previous QwQ (in thinking mode) and Qwen 2.5 instruct models (in non-thinking mode) on mathematics, code generation, and commonsense logical reasoning.
Expertise in agent capabilities, enabling precise integration with external tools in both thinking and unthinking modes and achieving leading performance among open-source models in complex agent-based tasks.
The first release includes Qwen3-235B-A22B MoE, Qwen3-30B-A3B MoE and Qwen3-32B dense models. All the models use Grouped Query Attention (GQA) and supports up to 128K context length with Yet another RoPE extensioN (YaRN).
Accelerating Qwen 3 with AMD: Key Benefits for Developers#
AMD Instinct GPU accelerators are purpose-built to handle the demands of next-gen models like Qwen 3:
MI300X can run Qwen3-235B-A22B MoE in BF16 with 4 GPUs and in FP8 with 2 GPUs and scale to 8 GPUs.
MI300X can run both Qwen3-30B-A3B MoE and Qwen3-32B dense in BF16 on a single GPU efficiently with full context length, minimizing GPU scaling overhead.
MI300X can serve 8 instances of Qwen3-30B-A3B MoE and Qwen3-32B dense to boost the throughput per node.
MI300X provides optimized inference with both vLLM and SGLang on Day 0 for developers to get started.
How to Run QWEN3 with vLLM on AMD Instinct GPUs#
Prerequisites:
Before you start, ensure you have access to AMD Instinct GPUs and the ROCm drivers set up.
Step 1. Launch docker container#
To run Qwen3 efficiently on MI300x, launch docker container as below
docker pull rocm/vllm:rocm6.3.1_instinct_vllm0.8.3_20250410
docker run -it \
--device /dev/dri \
--device /dev/kfd \
--network host \
--ipc host \
--group-add video \
--security-opt seccomp=unconfined \
-v $(pwd):/workspace \
rocm/vllm:rocm6.3.1_instinct_vllm0.8.3_20250410 /bin/bash
Step2. Start vLLM online server#
cd /workspace
VLLM_USE_TRITON_FLASH_ATTN=0 vllm serve qwen/Qwen3-30B-A3B --trust-remote-code &
Step3. Query the model with the following commands#
apt update && apt install jq -y
curl http://localhost:8000/v1/completions \
-H "Content-Type: application/json" \
-d '{ "model": "qwen/Qwen3-30B-A3B", "prompt": "San Francisco is", "max_tokens": 64, "temperature": 0 }' | jq ".choices[0].text"
The output will resemble the following example:
" a city of many neighborhoods, each with its own unique character and charm. From the foggy streets of the Marina to the vibrant energy of the Mission District, there's something for everyone. But if you're looking for a place that's both historic and trendy, you might want to check out the Haight-Ash"
Step 4. Additional test one can perform in the below example let us ask a math question (Optional)#
apt update && apt install jq -y
curl http://localhost:8000/v1/completions \
-H "Content-Type: application/json" \
-d '{ "model": "qwen/Qwen3-30B-A3B", "prompt": "solve the equation x^2-5x+6=0", "max_tokens": 640, "temperature": 0 }' | jq ".choices[0].text"
You can see the answer with the reasoning process below
"\nOkay, so I need to solve the quadratic equation x² - 5x + 6 = 0. Hmm, let me think. I remember that quadratic equations can often be solved by factoring, completing the square, or using the quadratic formula. Let me try factoring first because if it factors nicely, that might be the quickest way.\n\nThe equation is x² - 5x + 6 = 0. To factor this, I need two numbers that multiply to 6 (the constant term) and add up to -5 (the coefficient of the x term). Let me list the pairs of factors of 6:\n\n1 and 6\n2 and 3\n\nNow, considering the signs. Since the product is positive 6 and the sum is negative -5, both numbers must be negative. Because negative times negative is positive, and negative plus negative is negative. So let me check:\n\n-1 and -6: Their product is (-1)*(-6) = 6, and their sum is -1 + (-6) = -7. Hmm, that's not -5. Not quite.\n\n-2 and -3: Their product is (-2)*(-3) = 6, and their sum is -2 + (-3) = -5. Oh, there we go! That works.\n\nSo, the equation factors to (x - 2)(x - 3) = 0. Wait, let me check that. If I expand (x - 2)(x - 3), I get x² - 3x - 2x + 6 = x² - 5x + 6. Yep, that's exactly the original equation. Great, so factoring worked.\n\nNow, according to the zero product property, if the product of two factors is zero, then at least one of the factors must be zero. So, I can set each factor equal to zero and solve for x.\n\nFirst factor: x - 2 = 0. Adding 2 to both sides gives x = 2.\n\nSecond factor: x - 3 = 0. Adding 3 to both sides gives x = 3.\n\nSo, the solutions are x = 2 and x = 3. Let me verify these solutions by plugging them back into the original equation.\n\nFirst, x = 2:\n\nLeft side: (2)² - 5*(2) + 6 = 4 - 10 + 6 = 0. That works.\n\nNow, x = 3:\n\nLeft side: (3)² - 5*(3) + 6 = 9 - 15 + 6 = 0. That also works.\n\nSo both solutions check out. Alternatively, if I didn't factor it, I could use the quadratic formula. Let me try that method too to confirm.\n\nThe quadratic formula is x = [-b ± √(b² - 4ac)] / (2a). For the equation ax² + bx + c = 0. In this case, a = 1, b = -5,"
How to Run QWEN3 with SGLang on AMD Instinct GPUs#
Step 1. Running Qwen 3 with SGLang#
To run Qwen 3 with SGLang, let’s pull a pre-buillt docker
docker pull lmsysorg/sglang:v0.4.5.post1-rocm630
Step 2 Launch the docker container#
To run Qwen3 efficiently on MI300x, launch docker container as below
docker run -it \
--device /dev/dri \
--device /dev/kfd \
--network host \
--ipc host \
--group-add video \
--security-opt seccomp=unconfined \
-v $(pwd):/workspace \
lmsysorg/sglang:v0.4.5.post2-rocm630 /bin/bash
Step 3. Start SGLang server#
Once the container is launched , start the SGLang server
cd /workspace
python3 -m sglang.launch_server --model qwen/Qwen3-30B-A3B --trust-remote-code &
Step 4. Switch thinking and non-thinking mode#
Once the server is launched, try to switch the thinking and non-thinking mode of Qwen 3 by asking simple questions with ‘/think’ and ‘/no_think’. The model is in thinking mode by default.
# client_no_think.py
from openai import OpenAI
openai_api_key = "EMPTY"
openai_api_base = "http://localhost:30000/v1"
client = OpenAI(
api_key=openai_api_key,
base_url=openai_api_base,
)
chat_response = client.chat.completions.create(
model="qwen/Qwen3-30B-A3B",
messages=[
{"role": "system", "content": "You are a helpful assistant."},
{"role": "user", "content": "Tell me a joke. /no_think"},
]
)
print("Chat response:", chat_response.choices[0].message.content)
python client_no_think.py
The output will be short without any reasoning.
Chat response: <think>
</think>
Sure! Here's a joke for you:
Why don't skeletons fight each other?
Because they don't have the *guts*!
😄 Let me know if you want another!
Now switch it to thinking mode.
# client_think.py
from openai import OpenAI
openai_api_key = "EMPTY"
openai_api_base = "http://localhost:30000/v1"
client = OpenAI(
api_key=openai_api_key,
base_url=openai_api_base,
)
chat_response = client.chat.completions.create(
model="qwen/Qwen3-30B-A3B",
messages=[
{"role": "system", "content": "You are a helpful assistant."},
{"role": "user", "content": "Tell me a joke. /think"},
]
)
print("Chat response:", chat_response.choices[0].message.content)
python client_think.py
The response will include extended reasoning, demonstrating the model’s ability to articulate its thought process step by step, as shown below.
Chat response: <think>
Okay, the user asked for a joke. Let me think of a good one. Maybe something with a pun or wordplay. Let me recall some common joke structures. A pun on a common phrase or a play on words. How about something involving a cat? Wait, maybe a classic joke that's not too overused. Let me check... Oh, here's one: Why don't scientists trust atoms? Because they make up everything. Wait, that's a good one. But maybe the user has heard it before. Let me think of another. Or maybe a joke about a chicken crossing the road? But that's also classic. Let me see... How about a joke with a twist. Let's think of a setup and punchline. Maybe something about a bear? Why don't bears wear socks? Because they have bear feet. Hmm, that's a bit of a stretch. Or a joke about a duck. Why did the duck cross the road? To get to the other side. But that's too simple. Maybe a more original one. Let me try to create something. How about: Why did the math book look sad? Because it had too many problems. That's a classic. Oh well, maybe it's okay. Alternatively, a joke involving a library. Why did the library get a job? Because it wanted to borrow some time. No, that's not. Let me think again. Maybe something about an elephant. What do you call a fake noodle? An impasta. Oh, that's a good one. Or another one: Why did the scarecrow win an award? Because he was outstanding in his field. Wait, that's also classic. Maybe I should go with one of these. Let me check the user's possible response. If they need something more recent? Or maybe a different topic. Wait, the user said "Tell me a joke." So probably any joke is fine. Let me pick one that's a bit clever but not too obscure. The atomic one is solid. Or the math book one. Alternatively, maybe a joke with a twist. For example: What do you call it when a dog plays piano? A dog's piano. Or maybe that's too simple. Another idea: Why did the cookie go to the doctor? Because it was feeling crumbly. That's a play on words. I think that's good. Or another one: What do you call a fish wearing a bowtie? Sofishticated. Right, that's a pun. Maybe I should go with that. Let me confirm. The joke is: What do you call a fish wearing a bowtie? A sofishticated fish. Wait, no, the actual punchline is "A 'so fish-ticated' fish." Or maybe "A 'sophisticated' fish." But the pun is on 'sophisticated' and 'so fish.' So maybe that's a good one. Alternatively, the math book one. Let me check. Which one is more likely to be well-received? Maybe the math book joke. But I'm not sure. Let me think of another. Maybe a joke about a cat. Why did the cat jump on the computer? To chase the mouse. That's too simple. Or what do you call a bear with no teeth? A gummy bear. Oh, that's a classic. Maybe that's a good one. Alternatively, the fake noodle joke: What do you call a fake noodle? An impasta. That's also good. So the user asked for a joke. I should choose one that's clear and depends on the pun. Let's pick the one about the scarecrow. Why did the scarecrow win an award? Because he was outstanding in his field. That's a classic. Or the science one. Let me go with the science joke. Let me make sure it's correct. Because atoms make up everything. Yes. Alternatively, the library joke: Why did the library get a job? It wanted to check out some time. Wait, "check out some time" is double meaning, as in "check out" as in taking out books and time as in time management. But maybe that's not so common. Let's stick with the atoms joke. So the answer would be: Why don't scientists trust atoms? Because they make up everything. Alternatively, if the user wants a different one. But since they asked for any joke, this is a good one. Let me go with that.
</think>
Here's a joke for you:
Why don't scientists trust atoms?
Because they make up everything!
😄 Let me know if you'd like another!
This dynamic mode-switching feature is particularly valuable in multi-turn conversations, allowing users to toggle between thinking and non-thinking modes based on task requirements. Figure 1 below showcases a live demo of the Qwen 3 reasoning model operating on a single MI300X GPU, highlighting its efficiency and real-time performance.
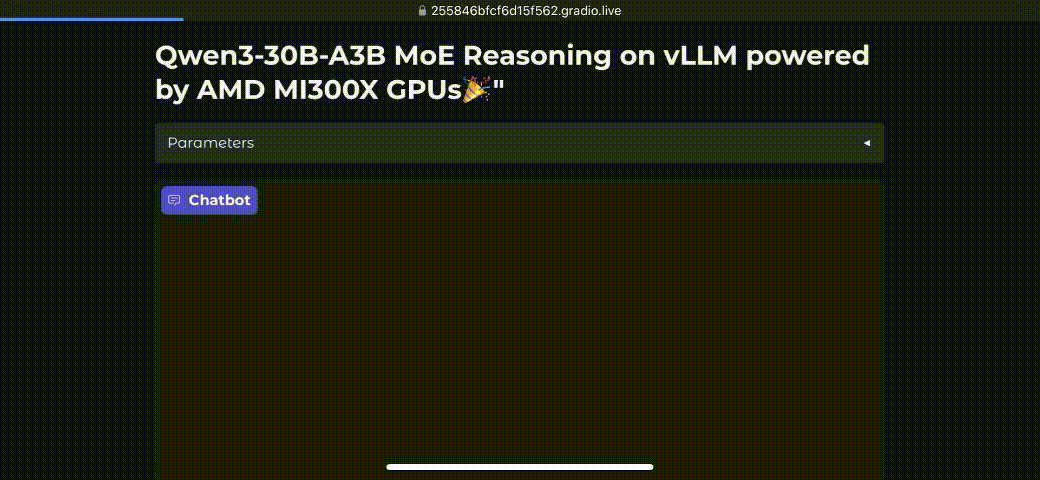
Figure 1. QWEN3 Demo on AMD MI300X#
Summary#
This blog provides a step-by-step Day 0 guide allowing you to explore the power of Alibaba’s Qwen 3 models on AMD Instinct MI300X GPUs. With both vLLM and SGLang supported on AMD Instinct GPUs, developers can immediately build and scale innovative AI applications including code generation, logical reasoning, and agent-based tasks. Stay tuned for more technical insights and updates on how AMD and our partners are advancing AI and optimizing Qwen 3 performance.
Acknowledgements#
AMD team members who contributed to this effort: Peng Sun, Jacky Zhao, Carlus Huang, Joe Shajrawi, Gregory, Shtrasberg, Anshul Gupta.
Additional Resources#
Visit the ROCm AI Developer for additional tutorials, blogs, open-source projects, and other resources for AI development on AMD GPUs.
Explore AMD ROCm Software https://www.amd.com/en/products/software/rocm.html
AMD Instinct Accelerators: https://www.amd.com/en/products/accelerators/instinct.html
Disclaimers#
Third-party content is licensed to you directly by the third party that owns the content and is not licensed to you by AMD. ALL LINKED THIRD-PARTY CONTENT IS PROVIDED “AS IS” WITHOUT A WARRANTY OF ANY KIND. USE OF SUCH THIRD-PARTY CONTENT IS DONE AT YOUR SOLE DISCRETION AND UNDER NO CIRCUMSTANCES WILL AMD BE LIABLE TO YOU FOR ANY THIRD-PARTY CONTENT. YOU ASSUME ALL RISK AND ARE SOLELY RESPONSIBLE FOR ANY DAMAGES THAT MAY ARISE FROM YOUR USE OF THIRD-PARTY CONTENT.