Boosting Llama 4 Inference Performance with AMD Instinct MI300X GPUs#
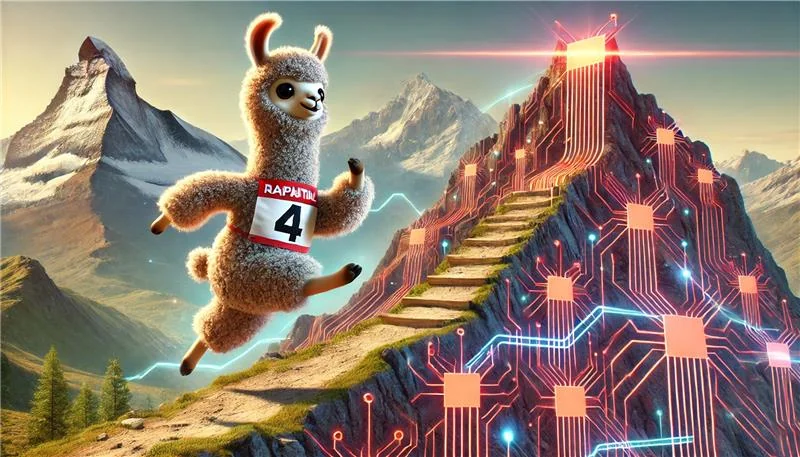
In our previous blog post, we explored how to deploy Llama 4 using AMD Instinct™ MI300X GPUs with vLLM. We also highlighted that MI300X and MI325X GPUs are capable of running the full 400B-parameter Llama 4 Maverick model in BF16 precision on a single node, significantly reducing infrastructure complexity. Their substantial HBM memory capacity further supports extended context lengths, enabling high throughput and efficient model execution.
In this blog we will now dive deeper into how using the vLLM framework, critical kernel optimizations like AI Tensor Engine for ROCm™, serving parameters from vLLM framework, and larger memory from MI300X GPUs collectively contribute to significant performance improvements.
Quick Overview of Llama 4 Architecture#
Meta released two models in its Llama 4 series: the Llama 4 Scout and the Llama 4 Maverick. Llama 4 Scout is a Mixture-of-Experts (MoE) model with 16 experts, with 109 billion total parameters, with 17 billion active per token. Llama 4 Maverick has 128 experts with 400 billion total parameters, with 17 billion active per token. This multi-modal architecture integrates both text and vision inputs. Text is embedded using token and positional embeddings, while images (336×336 RGB) are integrated in from 14X14 to 24×24 segments and passed through a 34-layer vision transformer with 16 attention heads and MLPs sized 1408→5632→1408. The resulting image and text embeddings are aligned via a multi-modal projector and sent to a 48-layer text decoder with 40 attention heads (head dimension 128), featuring a Mixture-of-Experts (MoE) with 16 out of 128 experts activated—one per token. The final output is generated from a vocabulary of 202,048 tokens. Figure 1 below provides an overview of the Llama 4 architecture.
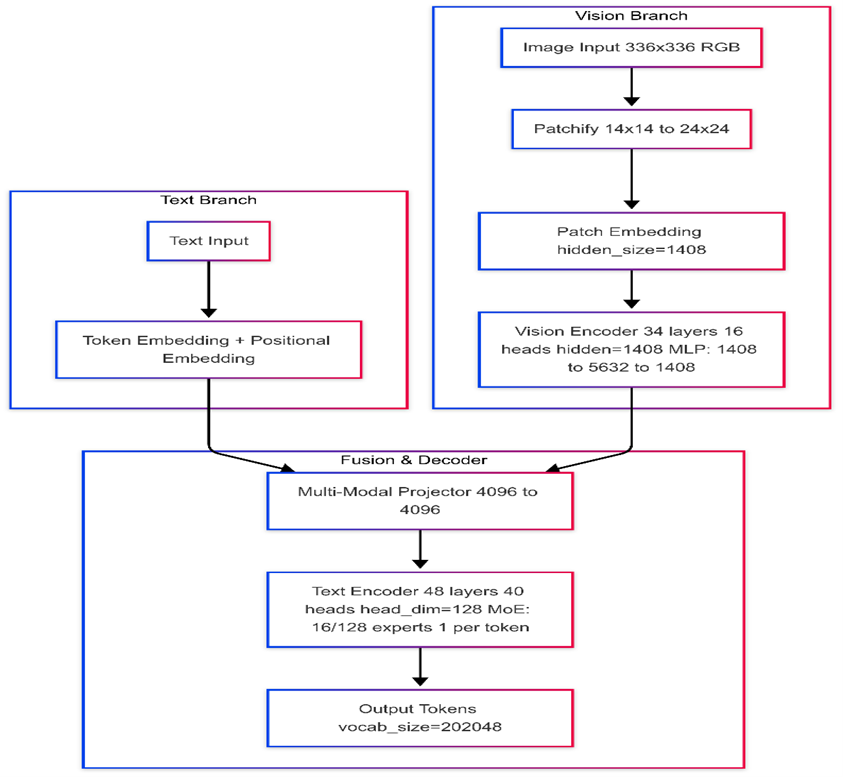
Figure 1. Llama 4 - architecture overview#
Key Takeaway#
Leveraging the latest vLLM framework, MI300X GPUs delivers 1.1X throughput performance boost as compared to the NVIDIA H200
AI Tensor Engine for ROCm software (AITER) kernels namely AITER block-scale fused MoE, AITER AllREDUCE and AITER attention kernel are optimized for Llama 4 contributing to performance boost on MI300X GPUs vs NVIDIA H200
vLLM serving parameters max_num_batched_tokens, max_model_len was a key to unlock and achieve the full potential of MI300X GPUs.
Performance Optimizations for Llama 4 on MI300X GPUs#
To achieve a 1.1× throughput improvement on MI300X GPUs compared to NVIDIA GPUs, we applied key kernel optimizations from the AI Tensor Engine (AITER) for ROCm™ and leveraged serving parameters from the vLLM framework. In the following sections, we detail the specific kernels and parameters employed.
AI Tensor Engine for ROCm (AITER)#
AMD introduced AITER, which is a centralized repository filled with high-performance AI operators designed to accelerate various AI workloads. By leveraging AITER’s advanced optimizations, Llama 4 kernels have been optimized on MI300X namely AITER block-scale fused MoE, AITER AllREDUCE, and AITER attention kernel. Here you can learn more about AITER and how to use AITER ROCm/aiter: AI Tensor Engine for ROCm and AITER: AI Tensor Engine For ROCm — ROCm Blogs. The integration of AITER in vLLM for the Llama 4 provides a competive edge for MI300X GPUs in total throughput(tokens/seconds).
vLLM Serving Parameters#
vLLM framework provides serving parameters used to configure an optimize how LLMs serve during inference. These parameters are critical knobs to fine tune LLM inferece based on application needs. We found that by tuning serving parameter like max_num_batched_tokens and max_model_len can improve maximum performance in our case throughput by controlling batch sizes and limiting the length of the prompt.
Llama 4 Serving Benchmark#
MI300X GPUs deliver competitive throughput performance using vLLM. Throughput, measured by total output tokes per second is a key metric when measuring LLM inference . As shown in Figure 2, MI300X GPUs delivers competitive performance under identical configuration as compared to Llama 4 using vLLM framework.
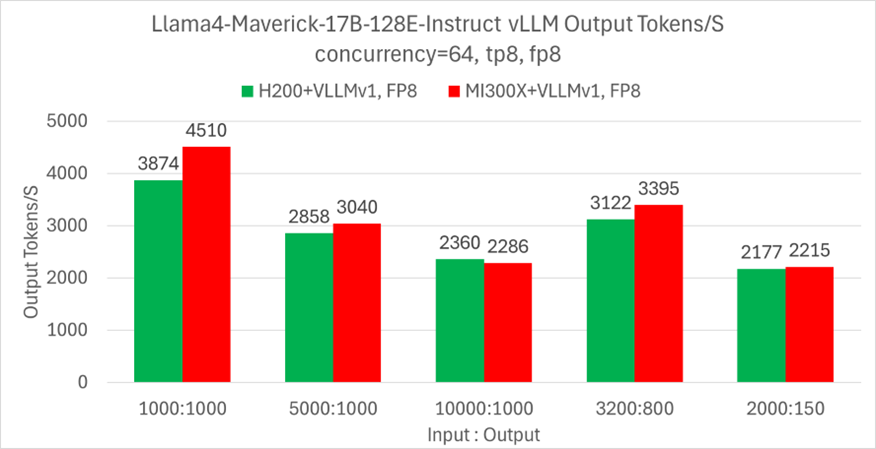
Reproducing Benchmark Results on Your System#
Now let’s reproduce the same performance results on your system and apply the same techniques to your application for optimal performance on MI300X GPUs. The following instructions assume that the user already downloaded a model. If not, Follow the steps mentioned here to download the models.
AMD Instinct MI300X GPUs with vLLM#
Set relevant environment variables and launch the AMD ROCm vLLM container.
docker pull rocm/vllm-dev:llama4-20250425
export MODEL_DIR="~/Llama-4-Maverick-17B-128E-Instruct-FP8"
docker run -it \
--ipc=host \
--network=host \
--privileged \
--shm-size 32G \
--cap-add=CAP_SYS_ADMIN \
--device=/dev/kfd \
--device=/dev/dri \
--group-add video \
--group-add render \
--cap-add=SYS_PTRACE \
--security-opt seccomp=unconfined \
--security-opt apparmor=unconfined \
-v $MODEL_DIR:/model \
rocm/vllm-dev:llama4-20250425
Start serving the model with vllm.
VLLM_USE_V1=1 \
VLLM_ROCM_USE_AITER=1 \
VLLM_WORKER_MULTIPROC_METHOD=spawn \
SAFETENSORS_FAST_GPU=1 \
vllm serve /model \
--disable-log-requests \
-tp 8 \
--no-enable-prefix-caching \
--max_num_batched_tokens 32768 \
--max-num-seqs 1024 \
--max-model-len 36000 &
Run the vLLM benchmark serving script with given parameters.
ISL_OSL=("2000:150" "1000:1000" "5000:1000" "10000:1000" "3200:800")
for in_out in ${ISL_OSL[@]}
do
isl=$(echo $in_out | awk -F':' '{ print $1 }')
osl=$(echo $in_out | awk -F':' '{ print $2 }')
vllm bench serve \
--model /model \
--dataset-name random \
--random-input-len $isl \
--random-output-len $osl \
--max-concurrency 64 \
--num-prompts 640 \
--ignore-eos \
--percentile_metrics ttft,tpot,itl,e2el
NVIDIA H200 GPU with vLLM#
Set the relevant environment variable and launch the Nvidia vllm container.
docker pull vllm/vllm-openai:v0.8.4
export MODEL_DIR="~/Llama-4-Maverick-17B-128E-Instruct-FP8"
docker run -it \
--ipc=host \
--network=host \
--privileged \
--shm-size 32G \
--gpus all \
-v $MODEL_DIR:/model \
vllm/vllm-openai:v0.8.4
Start the vLLM server:
SAFETENSORS_FAST_GPU=1 \
vllm serve /model \
--disable-log-requests \
-tp 8 \
--no-enable-prefix-caching \
--max-model-len 36000 &
Run the vLLM benchmark serving script with given parameters.
ISL_OSL=("2000:150" "1000:1000" "5000:1000" "10000:1000" "3200:800")
for in_out in ${ISL_OSL[@]}
do
isl=$(echo $in_out | awk -F':' '{ print $1 }')
osl=$(echo $in_out | awk -F':' '{ print $2 }')
vllm bench serve \
--model /model \
--dataset-name random \
--random-input-len $isl \
--random-output-len $osl \
--max-concurrency 64 \
--num-prompts 640 \
--ignore-eos \
--percentile_metrics ttft,tpot,itl,e2el
Summary#
Through a combination of AITER optimizations, fused kernel support, and fine-tuned vLLM parameters, Llama 4 inference on AMD MI300X GPUs delivers industry-leading throughput. As a result, the MI300X has emerged as a strong competitor to NVIDIA’s H200 for Llama 4 deployments. With the right software optimizations, MI300X GPUs achieve state-of-the-art throughput for both the Llama 4 Scout and Maverick models. Our benchmarks demonstrate that MI300X not only rivals, but in several cases surpasses, the performance of NVIDIA’s H200—particularly under higher concurrency and extended context lengths. These enhancements position MI300X GPUs as a highly competitive platform for large-scale LLM deployments.
References#
AITER Github: ROCm/aiter
vLLM Container for Inference and Benchmarking vLLM inference
AMD ROCm documentation AMD ROCm
Endnotes#
[1] On average, a system configured with an AMD Instinct™ MI300X GPUs shows 1.1x Llama 4 throughput performance boost using vLLM. Testing done by AMD on 04/28/2025, results may vary based on configuration, usage, software version, and optimizations.
SYSTEM CONFIGURATION#
AMD Instinct ™ MI300X GPU platform
System Model: Supermicro AS-8125GS-TNMR2
CPU: 2x AMD EPYC 9654 96-Core Processor
NUMA: 2 NUMA node per socket. NUMA auto-balancing disabled/ Memory:
2304 GiB (24 DIMMs x 96 GiB Micron Technology MTC40F204WS1RC48BB1 DDR5
4800 MT/s)
Disk: 16,092 GiB (4x SAMSUNG MZQL23T8HCLS-00A07 3576 GiB, 2x SAMSUNG
MZ1L2960HCJR-00A07 894 GiB)
GPU: 8x AMD Instinct MI300X 192GB HBM3 750W
Host OS: Ubuntu 22.04.4
System BIOS: 3.2
System Bios Vendor: American Megatrends International, LLC.
Host GPU Driver: (amdgpu version): ROCm 6.3.1\
NVIDIA HGX H200 Platform
System Model: Supermicro SYS-821GE-TNHR
CPU: 2x Intel Xeon Platinum 8592V 64-Core Processor
NUMA: 2 NUMA node per socket. NUMA auto-balancing enabled
Memory: 3072 GiB (32 DIMMs x 96 GiB Micron Technology
MTC40F204WS1RC56BB1 DDR5 5600 MT/s)
Disk: 432TiB (16 x 27TiB SOLIDIGM SBFPF2BU307T)
GPU: 8x NVIDIA Hopper H200 141GB HBM 700W
Host OS: Ubuntu 22.04.5
System BIOS: 2.1
System Bios Vendor: American Megatrends International, LLC.
Host GPU Driver: Cuda 12.5
Disclaimers#
Third-party content is licensed to you directly by the third party that owns the content and is not licensed to you by AMD. ALL LINKED THIRD-PARTY CONTENT IS PROVIDED “AS IS” WITHOUT A WARRANTY OF ANY KIND. USE OF SUCH THIRD-PARTY CONTENT IS DONE AT YOUR SOLE DISCRETION AND UNDER NO CIRCUMSTANCES WILL AMD BE LIABLE TO YOU FOR ANY THIRD-PARTY CONTENT. YOU ASSUME ALL RISK AND ARE SOLELY RESPONSIBLE FOR ANY DAMAGES THAT MAY ARISE FROM YOUR USE OF THIRD-PARTY CONTENT.