Beyond Text: Accelerating Multimodal AI Inference with Speculative Decoding on AMD Instinct™ MI300X GPUs#
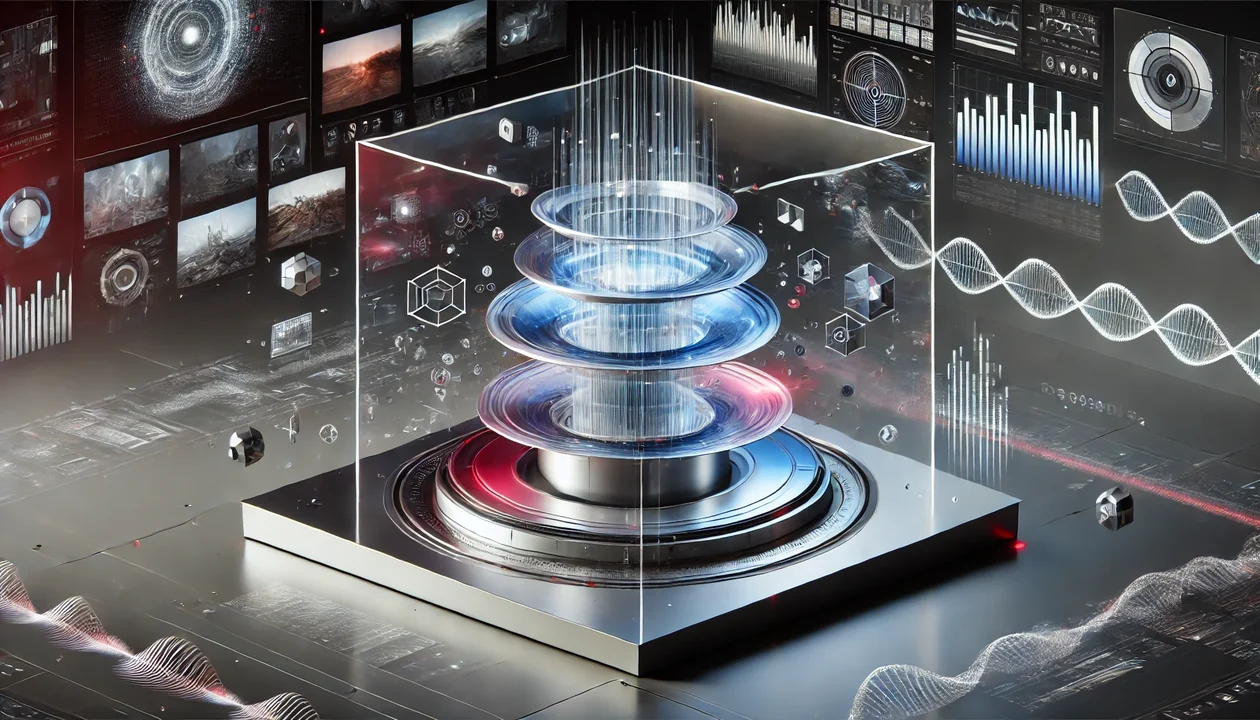
In the rapidly evolving landscape of artificial intelligence, multimodal models have emerged as powerful tools capable of processing and generating content across different modalities—text, images, audio, and more. Meta’s recent release of the multimodal Llama 4 models, including Llama 4 Scout and Llama 4 Maverick, exemplifies this advancement. Despite their impressive functionalities, such models face significant computational challenges, particularly in generation speed and resource efficiency due to a much larger context length compared to text-only models. Enter speculative decoding: a promising technique that has revolutionized text generation in large language models and is now finding exciting applications in multimodal contexts. Speculative decoding allows AI models to generate outputs faster by speculating several steps ahead and confirming predictions in fewer passes. In this blog you will learn, step-by-step, how speculative decoding can help you unlock significant inference speedups for multimodal systems while maintaining output quality using ROCm on AMD Instinct MI300X GPUs.
The Challenge of Multimodal Context Windows#
One of the most significant hurdles facing multimodal models is the substantially larger context windows required to process multimodal inputs. Unlike pure text models, multimodal models must handle dense information encoded in pixels, audio waveforms, or video frames alongside textual data, as it can be inferred from the overall architecture of multimodal models in Figure 1. This expanded context creates several critical bottlenecks:
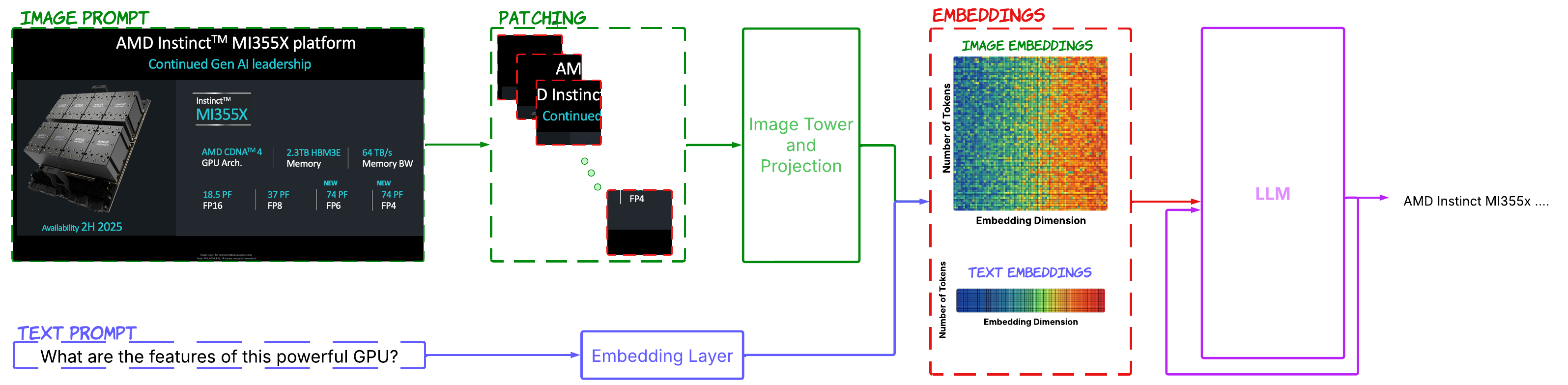
Figure 1. Overall architecture of multimodal models.#
Expanding Token Requirements#
When an image is tokenized and fed into a multimodal model, it typically consumes hundreds or even thousands of tokens - far more than its traditional textual LLMs would require. A single high-resolution image might be represented by thousands of tokens, while a video clip can easily demand tens of thousands of tokens. This explosion in token count directly impacts:
Attention Computation: The self-attention mechanism, central to transformer-based models, scales quadratically with sequence length, making computation exponentially more expensive as context grows.
Memory Utilization: Larger contexts require significantly more memory to store key-value caches.
Generation Latency: With larger contexts to process, each decoding step takes longer, creating noticeable delays in interactive applications.
Advances in Multimodal Speculative Decoding#
The elegance of speculative decoding lies in its ability to circumvent the inherent sequential nature of autoregressive generation. By “guessing” multiple future tokens and verifying them in parallel. This approach is particularly valuable for multimodal systems where each forward pass through the model requires processing in an extensive cross-modal context. When implementing speculative decoding for multimodal models, we have two primary architectural choices of text-only drafters or multimodal drafters. The multimodal drafters have a significantly higher acceptance length (average number of tokens accepted at each round of speculation and verification) than the text-only drafters, which boosts the overall speedups gained with these models despite their increased computational costs.
While speculative decoding has shown tremendous success in accelerating text-only language models, extending these techniques to multimodal domains presents unique and formidable challenges. Unlike text generation, where patterns and probabilities follow relatively consistent linguistic rules, multimodal systems must navigate the intricate relationships between visual elements, audio signals, and textual descriptions. Finding appropriate drafting models that perform well with high acceptance length and low latency is significantly more complex than in text-only speculative decoding. As you can see in Figure 2, the multimodal drafter, unlike a text-only drafter, can correctly draft tokens grounded in visual input, such as parameters or details that are visible in the image but not inferable from the text alone. Hence, it can achieve a much higher acceptance length overall.
In this blog, we’ll explore how speculative decoding techniques are being adapted to address these unique challenges of multimodal generation, offering promising paths to maintain the richness of multimodal understanding while improving generation speed up to 3x[1] in some tasks. This represents our first step toward comprehensive multimodal inference workload optimization, an area that remains largely unexplored and requires greater attention from the AI research community. Our codebase, which is based on the PyTorch GPTFast repo, for reproducing the results and developing more models will be released here.
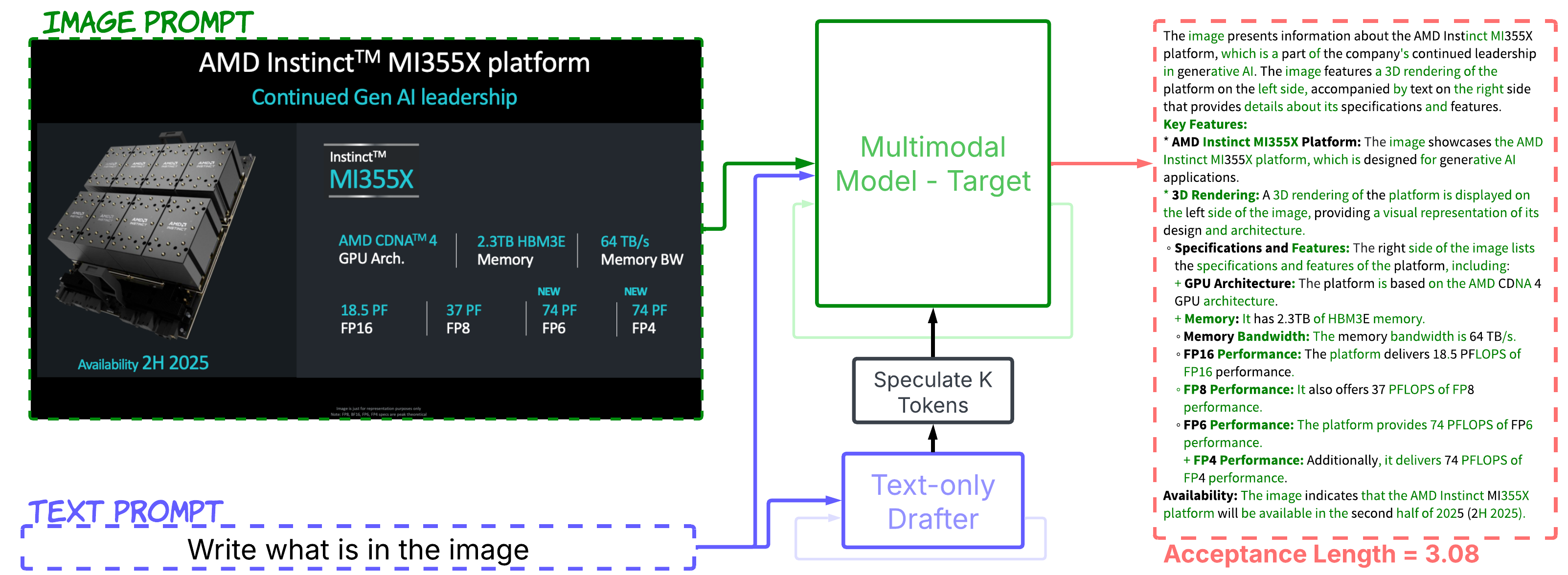
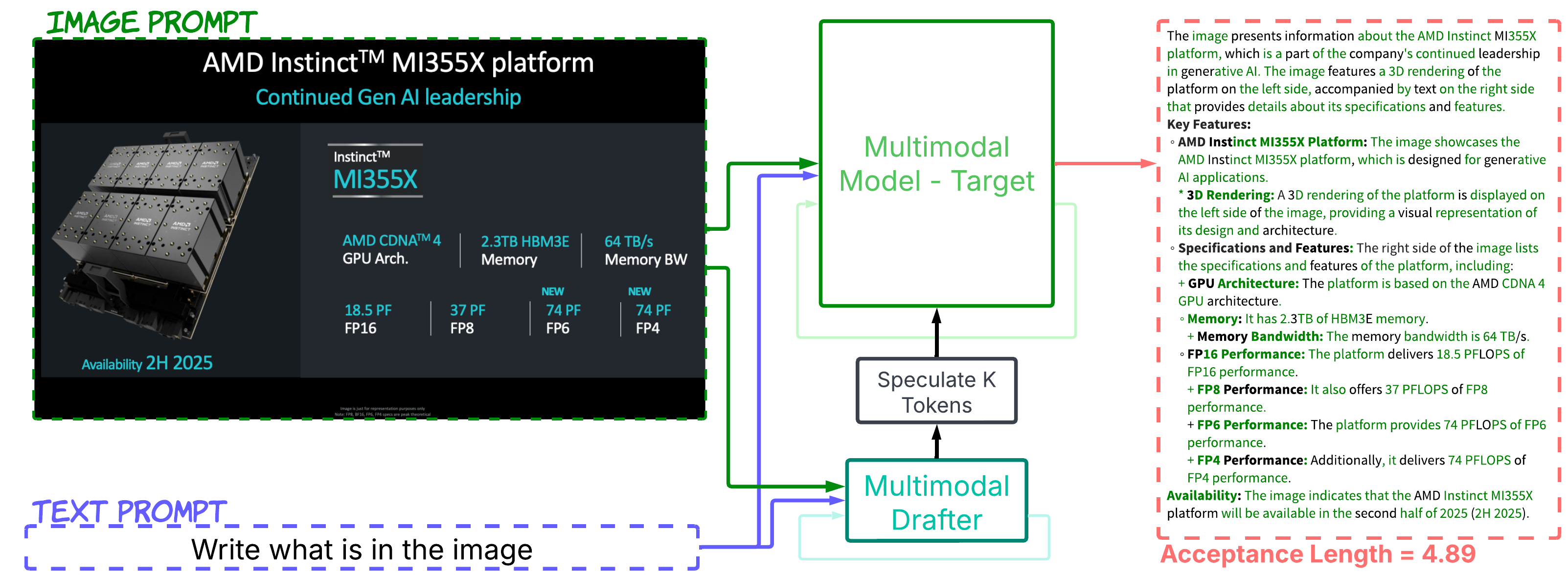
Figure 2. Comparative performance of text-only vs. multimodal drafters in speculative decoding. Green tokens represent successfully speculated tokens. The multimodal drafter demonstrates superior token speculation accuracy, particularly for image-embedded text, resulting in a significantly higher token acceptance length compared to the text-only drafter. For instance, the multimodal drafter can speculate correctly the first instance of “AMD Instinct” from the image, while the text-only drafter fails to do so.#
Key Takeaways#
Multimodal drafters outperform text-only drafters - Multimodal drafters consistently achieve higher acceptance lengths than text-only drafters, leading to better overall performance despite slightly higher computational costs. The ability to access visual information during speculation gives them a significant advantage.
Task-specific performance varies significantly - Tasks involving structured visual data interpretation (like accounting and finance) achieve the highest speedups (up to 3x), while more subjective analysis tasks (like design and art theory) show lower improvements. OCR-heavy applications benefit most from multimodal speculative decoding.
Visual token compression offers optimization opportunities - Selectively reducing visual token dimensionality during drafting can accelerate the speculative process while maintaining the target model’s original probability distribution, addressing the challenge of managing large multimodal context windows.
Model Families#
For our experiments on speculative decoding for multimodal models, we focused on two distinct architectural families that represent the current state-of-the-art approaches to multimodal integration:
Early Fusion Models#
Early fusion models adopt a straightforward but effective approach to multimodal integration:
Visual data passes through a specialized visual encoder (typically a vision transformer)
The resulting visual features are projected into the same embedding space as text
These projected visual embeddings are concatenated with text embeddings
The combined sequence is processed by a standard language model architecture
This architecture treats visual tokens essentially as a prefix to the textual sequence, allowing the model to attend to both modalities through the same self-attention mechanisms. For our experiments with early fusion, we selected two prominent models:
QWEN 2.5 VL: The latest multimodal variant of the QWEN family, featuring enhanced visual understanding capabilities and strong performance on vision-language benchmarks.
Llava-NeXT: Built on the QWEN 2 architecture, Llava-NeXT represents a cutting-edge approach to early fusion with optimizations for efficient processing of mixed visual-textual inputs.
Early fusion models typically excel at tasks requiring tight integration between modalities, as all tokens (visual and textual) can directly attend to each other throughout the network.
Intermediate Fusion Models#
Intermediate fusion takes a more sophisticated approach to cross-modal integration:
Visual data is processed through its dedicated encoder and projected into a compatible representation
However, instead of direct concatenation, the visual embeddings remain separate
Cross-attention mechanisms are introduced at specific layers of the network
These cross-attention layers allow textual representations to attend to visual features without merging the sequences
For our experiments with intermediate fusion architectures, we focused on:
Llama 3.2 Vision: The vision-enabled variant of the Llama 3.2 family, which implements an elegant intermediate fusion approach with carefully designed cross-attention mechanisms.
Intermediate fusion models often demonstrate advantages in preserving modality-specific processing while still enabling rich cross-modal reasoning. This architecture can be particularly effective for queries that require referencing specific visual elements while maintaining a primarily language-driven response generation.
By evaluating speculative decoding across both architectural families, our blog aims to identify whether certain fusion approaches are more amenable to acceleration through drafting techniques, and whether the effectiveness of text-only versus multimodal drafters varies depending on the underlying model architecture.
Experimental Results#
Our experiments systematically compare different drafting strategies across multiple model architectures and scales. We evaluate both early fusion models (QWEN 2.5 VL and Llava-NeXT) and intermediate fusion models (Llama 3.2 Vision), paired with drafters of various sizes and modalities. For each configuration, we measure key performance metrics including token acceptance length and generation speedups to provide a comprehensive understanding of the tradeoffs involved. Our experimental results are built around the Massive Multi-discipline Multimodal Understanding (MMMU) benchmark—a challenging dataset specifically designed to evaluate multimodal models across diverse domains.
Comparing Text vs. Multimodal Drafters#
In our comparison of multimodal drafters versus text-only drafters, we observed that multimodal drafters consistently achieve higher acceptance lengths than their text-only counterparts. This advantage stems from their ability to access and incorporate multimodal information during the speculation phase. The key trade-off emerges between the multimodal model’s superior acceptance length and the text drafter’s greater speed (resulting from the absence of vision processing components and shorter context lengths). Based on our analysis in figures 3 and 4 (below), we conclude that in most practical scenarios, multimodal models offer a more advantageous position in this trade-off, delivering better overall performance despite the slightly higher computational cost. Our experiments with both Qwen 2.5 VL and Llava OneVision (based on Qwen 2) models demonstrate that multimodal drafters attain significantly higher acceptance lengths than their text-only counterparts, which translates to proportionally higher speedups. However, the speedup percentage does not fully match the acceptance length improvement due to the computational overhead described in the trade-off.
Setup:
QWEN 2.5 VL (72B) with:
QWEN 2.5 VL (3B) as multimodal drafter
QWEN 2.5 (3B) as the text drafter
Llava-OneVision (72B) with:
Llava-OneVision (0.5B) as multimodal drafter
QWEN 2 Text (0.5B) as text-only drafter
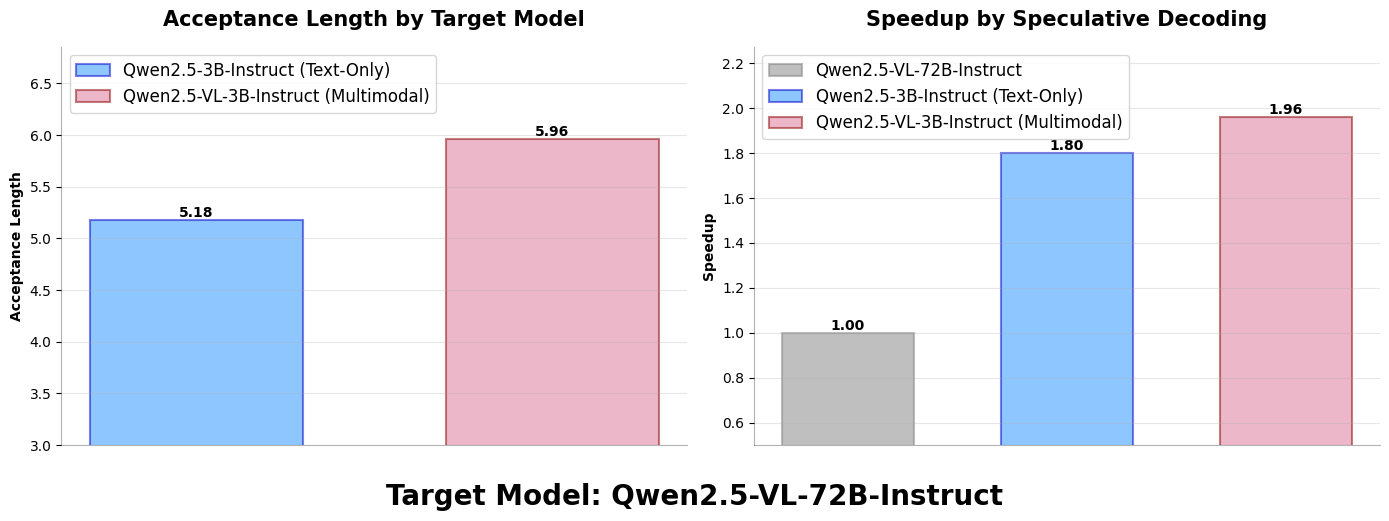
Figure 3. Acceptance length and speedup improvement of the multimodal drafter in Qwen model family over the text-only drafter#
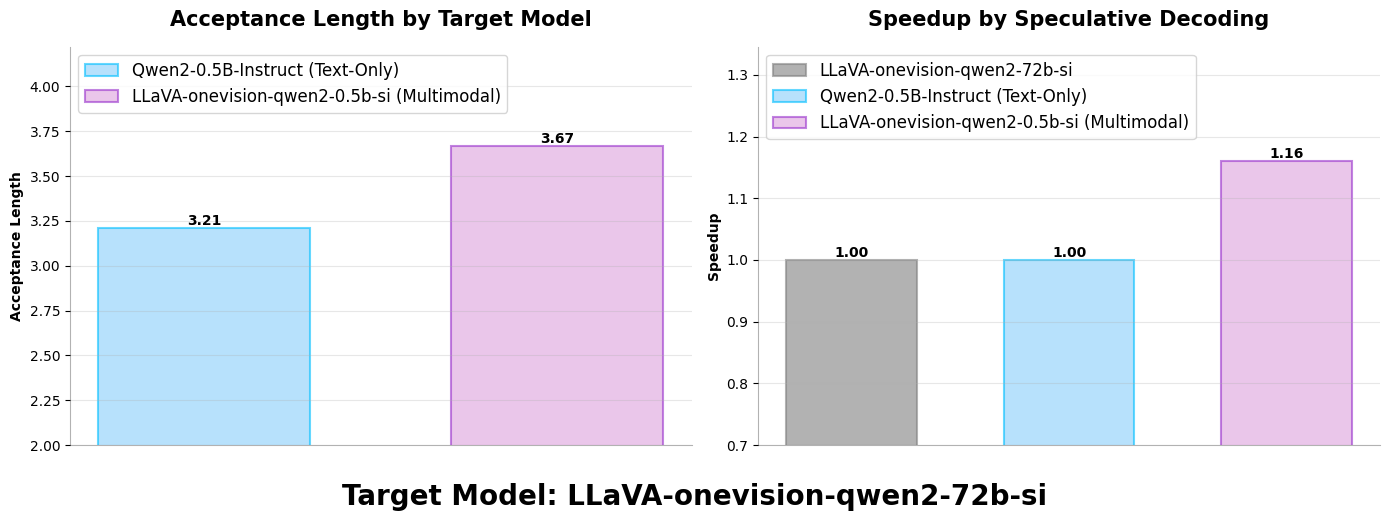
Figure 4. Acceptance length and speedup improvement of the multimodal drafter in Llava-OneVision model family over the text-only drafter#
Drafter Size Impact#
A key factor influencing both speedup and acceptance length in speculative decoding is the drafter size. Our experiments on multimodal models in Figure 5 confirm that larger drafters deliver substantially better speculation performance with higher acceptance lengths, while still maintaining efficient processing times. The experimental results demonstrate that increasing drafter size produces substantial improvements in acceptance length. For example, with Llava-OneVision models, upgrading from a 0.5B drafter to a 7B drafter increases the acceptance length from 3.67 to 5.6—a remarkable enhancement. This significant boost in acceptance length translates to a great speedup gains that outweigh the modest additional latency from the larger drafter.
Setup:
Llava-OneVision (72B) with multimodal drafters:
Llava-OneVision (7B) as multimodal drafter
Llava-OneVision (0.5B) as multimodal drafter
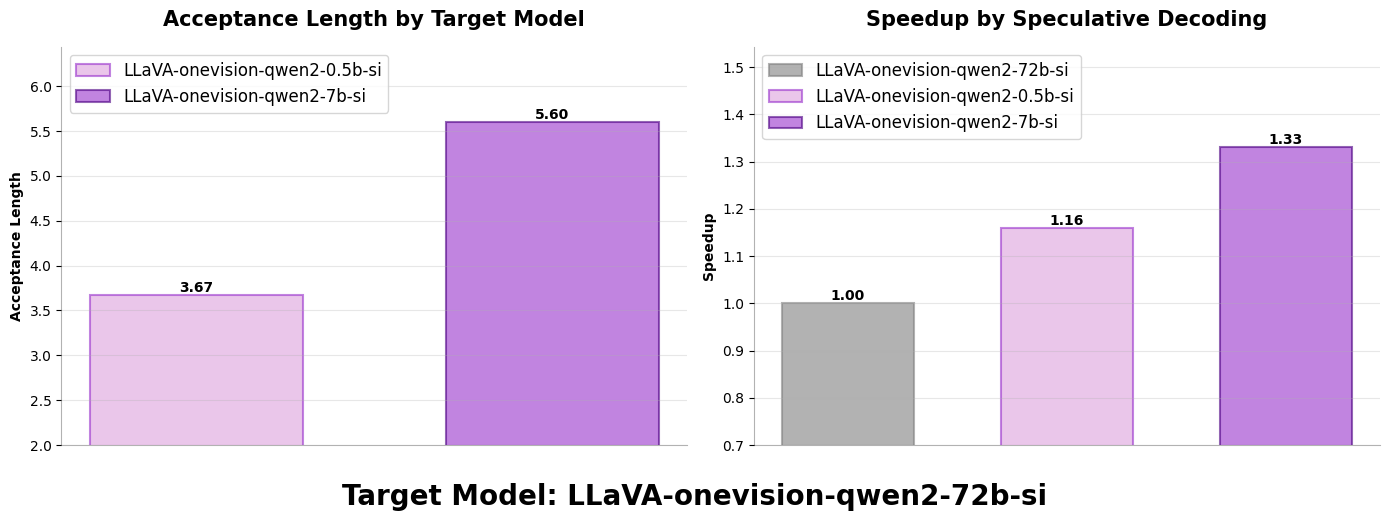
Figure 5. Increasing the size of the model can significantly increase the acceptance length of speculation, and improve the speedup gained from it#
Task-Specific Speedups in Multimodal Inference#
We evaluated speculative decoding performance across various tasks in the MMMU benchmark using Qwen 2.5 VL models, measuring both speedup and acceptance length for each task category. The results in Figure 6 reveal a clear pattern: tasks requiring structured visual data interpretation—such as accounting and finance, which often involve analyzing financial tables and generating formatted responses (in markup or LaTeX)—achieve the highest acceptance lengths and speedups. The accounting task, for example, demonstrates an impressive 3x acceleration. Conversely, tasks centered on subjective analysis and descriptive content—like design and art theory, which typically involve general discussions about well-known images—show comparatively lower speedup rates. These findings support our conclusion that tasks where critical response information is directly embedded in multimodal data, particularly OCR-heavy applications, benefit most substantially from multimodal speculative decoding.
Setup:
QWEN 2.5 VL (72B) with:
QWEN 2.5 VL (3B) as multimodal drafter
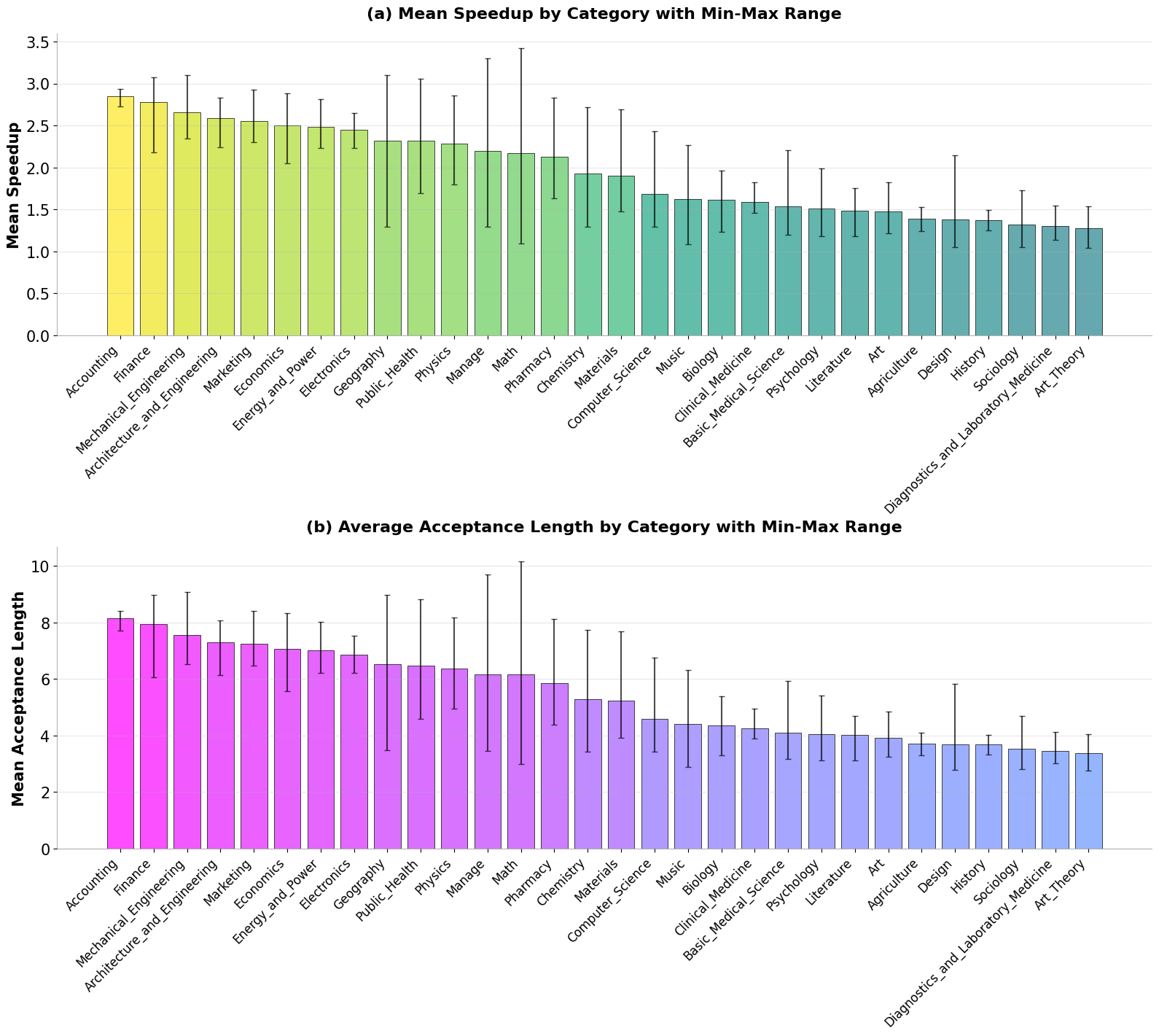
Figure 6. Task-specific speculative decoding performance across MMMU benchmark subcategories using the Qwen 2.5 VL model family. Tasks involving structured visual text interpretation, particularly accounting and finance, demonstrate exceptional acceleration—reaching up to 3× speedup—due to the multimodal drafter’s enhanced ability to predict tokens in OCR-heavy contexts. (a) Mean speedup across tasks, with minimum and maximum values indicated. (b) Average acceptance length per task in the dataset, also showing minimum and maximum values.#
Visual Token Compression in Drafter#
Managing multimodal context length in multimodal models presents a significant challenge that directly impacts generation speed and memory consumption. Research demonstrates that these tokens frequently contain high levels of sparsity and redundancy, resulting in inefficient resource utilization and slower generation times. In our Speculative Decoding framework, we maintain the target model’s performance while strategically applying these insights to optimize our drafter model. By selectively reducing visual token dimensionality during the drafting phase, we accelerate the speculative process, despite the resulting decrease in the drafter model’s acceptance length. Our experimental results in Figure 7 confirm that this pruning approach generally produces substantial overall speedups, particularly because the pruning occurs exclusively at the draft level, allowing the primary target model to maintain its original generation probability distribution.
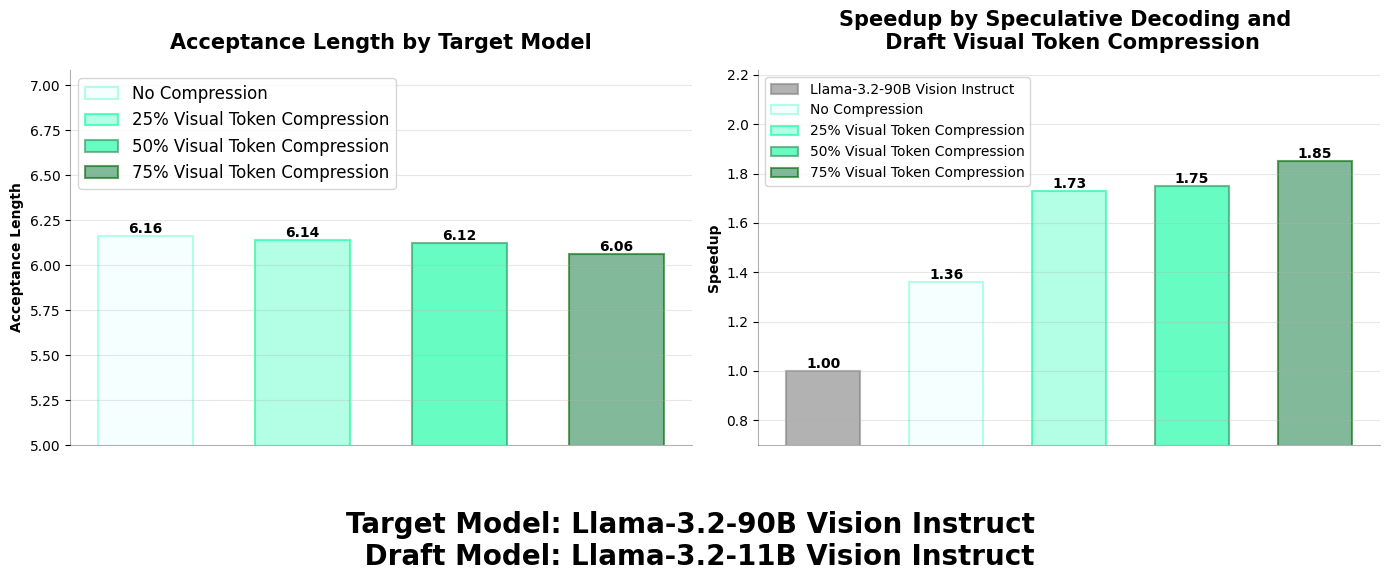
Figure 7. Impact of visual token dimensionality reduction on speculative decoding performance. Strategic pruning of visual tokens reveals inherent sparsity in image representations, enabling more efficient multimodal inference by accelerating the drafting process while maintaining the target model’s generative probability distribution.#
Accelerate Your Multimodal AI Implementations#
We’re excited to release our comprehensive codebase that makes multimodal speculative decoding accessible and easy to implement. If you’re working on vision-language models and looking to optimize inference performance, our codebase provides everything you need to get started.
Quick Start Guide#
First install PyTorch according to the instructions specific to your operating system. For AMD GPUs, we strongly recommend using ROCm™ software dockers like rocm/pytorch.
Clone the repository
git clone https://github.com/AMD-AIG-AIMA/gpt-fast
cd gpt-fast
Install dependencies
pip install -r requirements.txt
Explore Implemented Models: Our codebase includes ready-to-use implementations of multimodal models used in this blog:
QWEN 2.5 VL
Llava-OneVision
Llama 3.2 Vision
And many more text models
Performance Benchmarking: You can use our benchmarking code or use the included Gradio app designed for speculative decoding. For running the app use the following commands:
bash scripts/prepare.sh <target_model/repo_id> <download_dir>
bash scripts/prepare.sh <draft_model/repo_id> <download_dir>
python app.py --checkpoint_path <download_dir/target_model/repo_id> --draft_checkpoint_path <download_dir/draft_model/repo_id>
where <target_model/repo_id>
is the model id in the Huggingface library.
You can try the code for yourself → Access the Codebase. Contributions to this project are welcome.
Summary#
Our work on multimodal speculative decoding represents a significant advancement in accelerating inference for vision-language models, addressing the unique challenges posed by large multimodal context windows. Through extensive experimentation with both early fusion (QWEN 2.5 VL, Llava-NeXT) and intermediate fusion (Llama 3.2 Vision) architectures, we demonstrate that multimodal drafters consistently outperform text-only alternatives despite their slightly higher computational cost.
The results reveal compelling task-specific performance variations, with structured visual data interpretation tasks achieving remarkable speedups of up to 3× on some of tasks in the MMMU benchmark. We also show that strategic visual token compression during the drafting phase can further enhance performance while maintaining the target model’s probability distribution. Our findings establish a foundation for comprehensive multimodal inference optimization—an area that remains largely unexplored in AI research.
Our PyTorch-based implementation, built on the GPTFast repository, provides developers with accessible tools to implement these techniques in their own applications. We invite the developer community to explore our codebase, which offers seamless integration of the models discussed in this blog. As we continue to refine these methods, we look forward to seeing how speculative decoding transforms multimodal AI inference across diverse applications and domains.
SYSTEM CONFIGURATION#
AMD Instinct™ MI300X platform
Cloud: Tensorwave Inc
CPU: 2x AMD EPYC 9654 96-Core Processor
GPU: 8x AMD Instinct MI300X 192GB HBM3 750W
Host OS: Ubuntu 22.04.4
Host GPU Driver: (amdgpu version): ROCm 6.3.1
Disclaimers#
Third-party content is licensed to you directly by the third party that owns the content and is not licensed to you by AMD. ALL LINKED THIRD-PARTY CONTENT IS PROVIDED “AS IS” WITHOUT A WARRANTY OF ANY KIND. USE OF SUCH THIRD-PARTY CONTENT IS DONE AT YOUR SOLE DISCRETION AND UNDER NO CIRCUMSTANCES WILL AMD BE LIABLE TO YOU FOR ANY THIRD-PARTY CONTENT. YOU ASSUME ALL RISK AND ARE SOLELY RESPONSIBLE FOR ANY DAMAGES THAT MAY ARISE FROM YOUR USE OF THIRD-PARTY CONTENT.