Optimized ROCm Docker for Distributed AI Training#
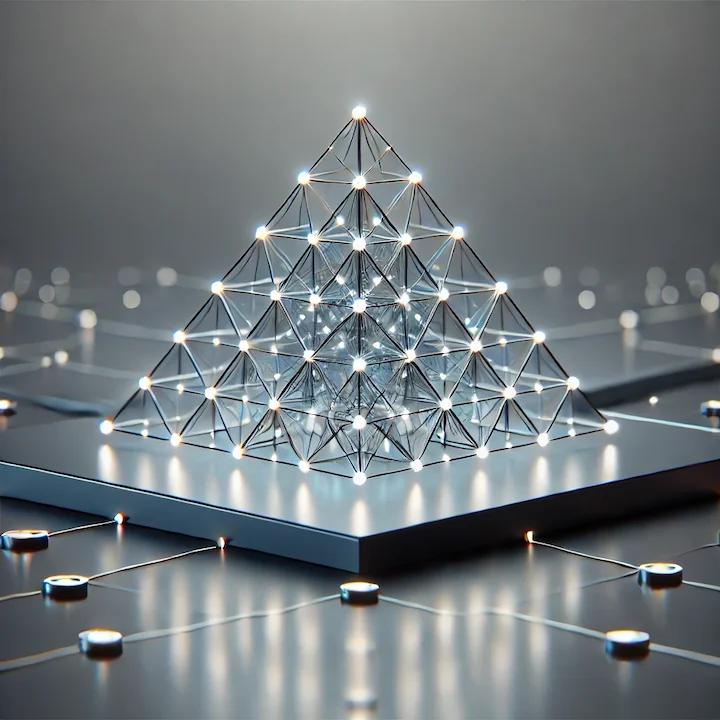
This blog will introduce you to the updated AMD Docker image, specifically built and optimized for distributed training. As you will see, the optimized AMD ROCm Docker image makes training large AI models faster and more efficient. It includes updates such as better fine-tuning tools, improved performance for multi-GPU setups, and support for FP8 precision, which helps speed up training while using less memory, and can provide you with an overall smoother and more efficient training experience on popular models such as Flux and Llama 3.1 running on AMD GPUs.
The blog will provide you with an in-depth overview of the recent updates focusing on enhanced scalability and efficiency within training pipelines. We will discuss the updated Docker images incorporating torchtune finetuning capability ,FP8 datatype support, single node performance boost, bug fixes and updated benchmarking scripts, ensuring a stable, consistent and reliable environment for managing complex distributed training workflows.
The PyTorch and Megatron-LM Training Dockers#
The ROCm™ PyTorch training docker release (v25.3) contains two updated Docker images for distributed training: PyTorch and Megatron-LM. Let’s briefly discuss their major features:
PyTorch Training Docker#
The ROCm Pytorch Training docker container provides a prebuilt, optimized environment for fine tuning, pre-training a model on AMD Instinct™ MI300X and MI325X GPUs.
Key Highlights
Updated benchmarking scripts for pre-training popular models such as Flux, Llama 3.1 8B, and Llama 3.1 70B.
Support for torchtune finetuning capability to enable full weight Low Ranking Adaption( LoRA) finetuning.
Added Huggingface Accelerate and torchtitan libraries to optimize the training with FSDP.
Integrated with MAD system for regression testing, documentation and example publication.
Megatron-LM Training Docker#
The ROCm Megatron-LM training docker is designed to enable efficient training of large-scale language models on AMD Instinct MI300X and MI325X GPUs. AMD Megatron-LM delivers enhanced scalability, improved performance and resource utilization for AI workloads. It is purpose-built to support models like Meta’s Llama 2, Llama 3, and Llama 3.1, enabling developers to train next-generation AI models with greater efficiency.
Key Highlights
The Megatron-LM focused Docker image is built on top of the Pytorch training Docker.
Supports multimode training, DeepseekV2 Lite and FP8 datatypes.
Finetuning with Torchtune#
Using an updated Docker equipped with optimized torchtune, users can have a streamlined out-of-box experience of finetuning, both full weights and LoRA, from the AMD Pytorch training docker release. The docker image is available in the torchtune dockerhub repository. Below is an example of using the PyTorch training Docker release v25.3 to run finetuning.
Launch PyTorch Training Docker
docker pull rocm/pytorch-training:v25.3
docker run -it --device /dev/dri --device /dev/kfd --network host --ipc host --group-add video --cap-add SYS_PTRACE --security-opt seccomp=unconfined --privileged -v $HOME:$HOME -v $HOME/.ssh:/root/.ssh --shm-size 64G --name training_env rocm/pytorch-training:v25.3
Clone ROCm MAD benchmarking repo
git clone https://github.com/ROCm/MAD
cd MAD/scripts/pytorch-train
Download models and dataset
# pass your HF_TOKEN
export HF_TOKEN=$your_personal_hf_token
./pytorch_benchmark_setup.sh
Lama 3.1 70B Full weight finetuning with Wikitext database
./pytorch_benchmark_report.sh -t finetune_fw -p BF16 -m Llama-3.1-70B
With larger HBM memory, MI300X GPUs can execute finetuning with larger batch sizes and do not rely on CPU offload, both benefit the training throughputs. This resulted in about 1.09x better unmasked token/sec/GPU in comparing to an equivalent H100 solution as shown in Figure 1 below. We observed that H100 runs out of memory in scenarios when CPU offload is turned off or for batch size 64 or larger.
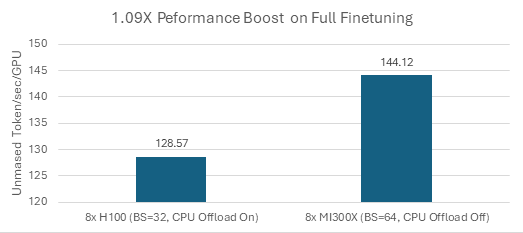
Figure 1: Full Fine-tuning Unmasked Tokens/sec/gpu w/ variable sample length from WikiText [1]#
Low Precision FP8 Multi-node Scaling Performance#
FP8 support is now integrated into the Megatron-LM docker container. Using the latest optimizations and configurations, found in the Megatron-LM docker container, our testing demonstrates 97% near-linear multi-node scaling, as illustrated in Figure 2. In other words, these benchmarks demonstrate minimal impacts of multi-node distributed training on GPU performance.
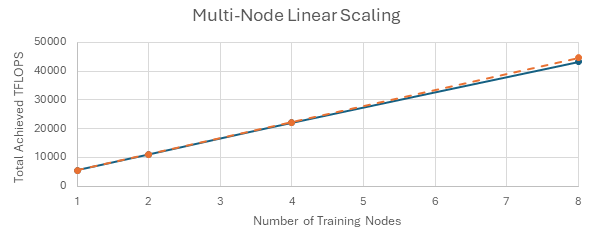
MoE support with Megatron-LM training#
AMD also offers support for Mixture of Experts (MoE) models—a class of deep learning architectures that leverage multiple specialized sub-models, or “experts.” MoE models dynamically route input data to the most relevant experts, enabling the scaling of model capacity while maintaining computational efficiency by activating only a subset of experts per input.
We observe that a single node (8x MI300X) delivers about 1.29x better performance vs. single node(8x H100) on DeepSeekV2-Lite training. We also now have an added advantage of being able to train the full model without checkpoint recompute to support larger micro batch size as shown in Figure 3.
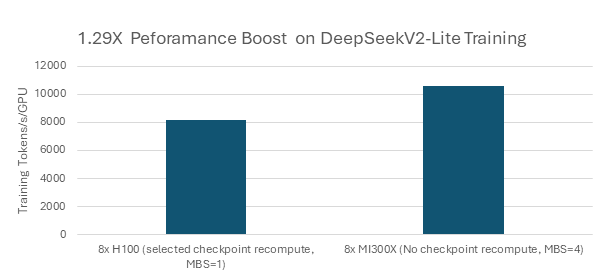
Summary#
AMD is committed to releasing an improved performance dockers at a regular cadence. This blog lays the foundation for an improved optimized docker for training with AMD GPUs. We are excited to continue and provide members of the open-source community with an opportunity to try our updated docker image for their training workloads. Stay tuned for more updates in our next blog as we’re working on implementing performance improvements, additional features and enhancing the user experience for Docker deployments moving forward.
Additional Resources#
Torchtune (AMD-AIG-AIMA/torchtune)
PyTorch training benchmark (ROCm/MAD)
MoE support with Megatron-LM docker using DeepSeekV2-Lite (ROCm/MAD)
Disclaimers#
Third-party content is licensed to you directly by the third party that owns the content and is not licensed to you by AMD. ALL LINKED THIRD-PARTY CONTENT IS PROVIDED “AS IS” WITHOUT A WARRANTY OF ANY KIND. USE OF SUCH THIRD-PARTY CONTENT IS DONE AT YOUR SOLE DISCRETION AND UNDER NO CIRCUMSTANCES WILL AMD BE LIABLE TO YOU FOR ANY THIRD-PARTY CONTENT. YOU ASSUME ALL RISK AND ARE SOLELY RESPONSIBLE FOR ANY DAMAGES THAT MAY ARISE FROM YOUR USE OF THIRD-PARTY CONTENT.