Applications & Models - Page 3#
Explore the latest blogs about applications and models in the ROCm ecosystem, including machine learning frameworks, AI models, and application case studies.
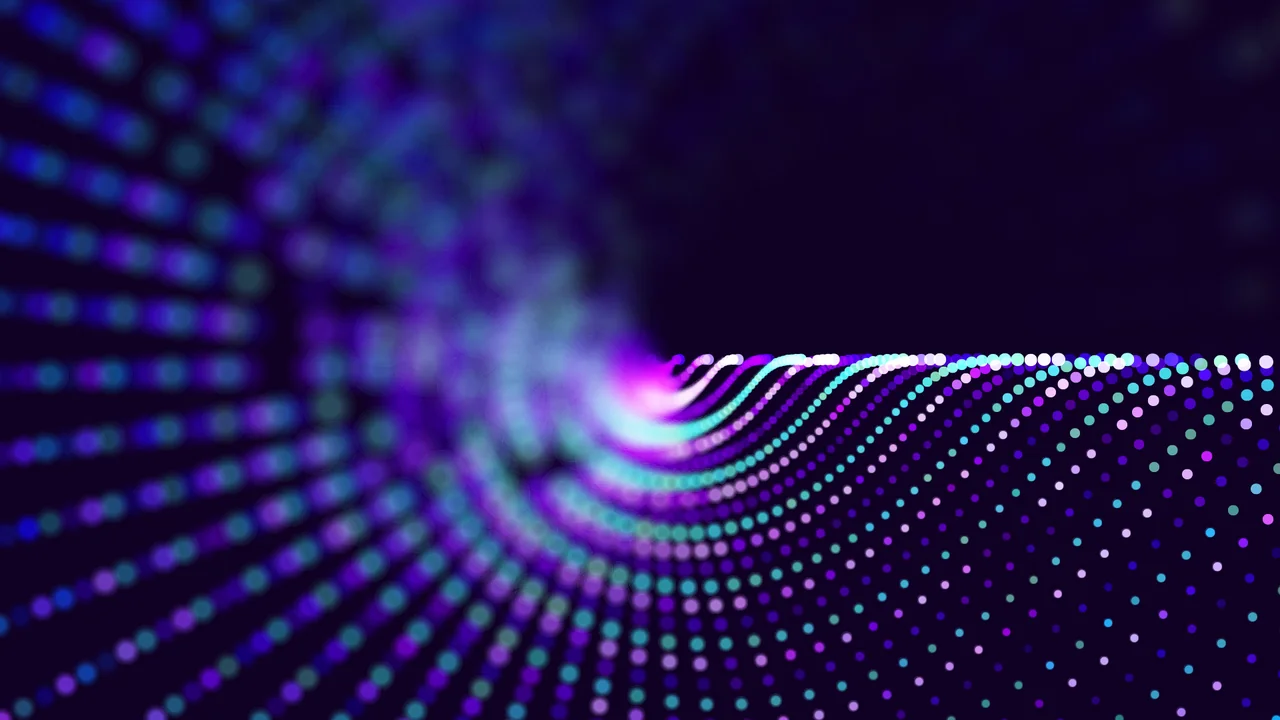
Distributed Data Parallel Training on AMD GPU with ROCm
This blog demonstrates how to speed up the training of a ResNet model on the CIFAR-100 classification task using PyTorch DDP on AMD GPUs with ROCm.
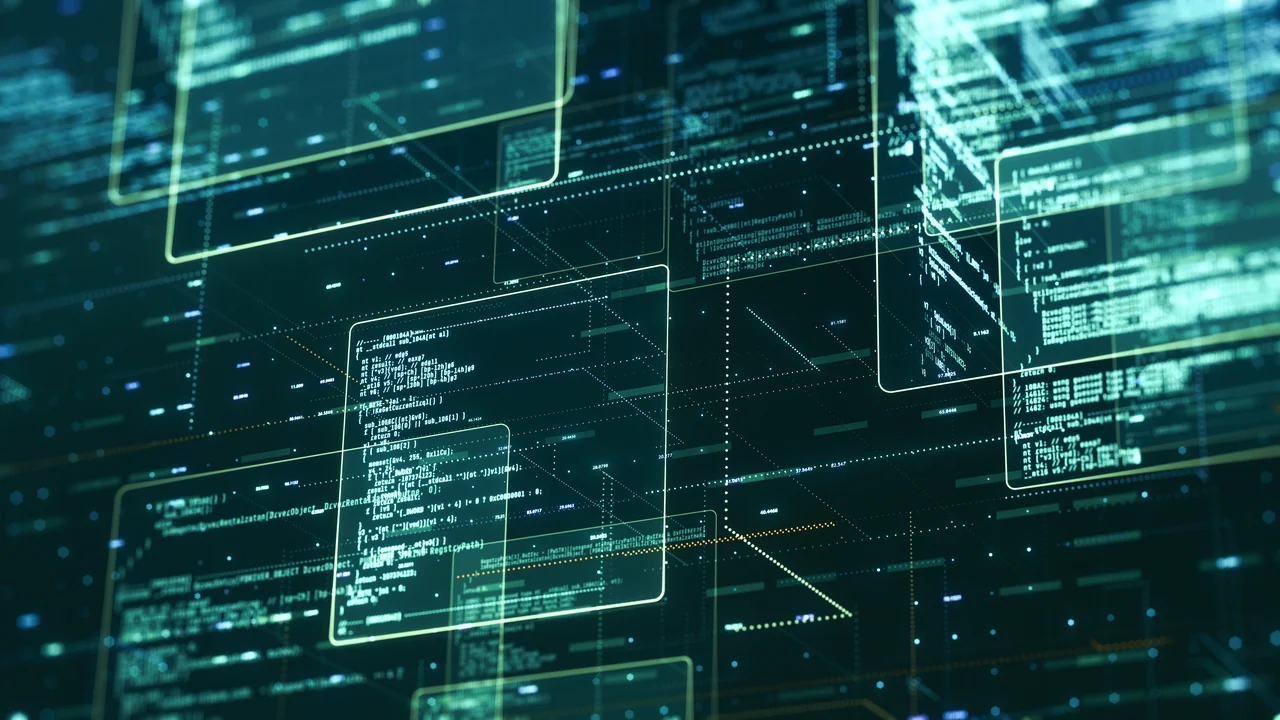
Torchtune on AMD GPUs How-To Guide: Fine-tuning and Scaling LLMs with Multi-GPU Power
Torchtune is a PyTorch library that enables efficient fine-tuning of LLMs. In this blog we use Torchtune to fine-tune the Llama-3.1-8B model for summarization tasks using LoRA and showcasing scalable training across multiple GPUs.
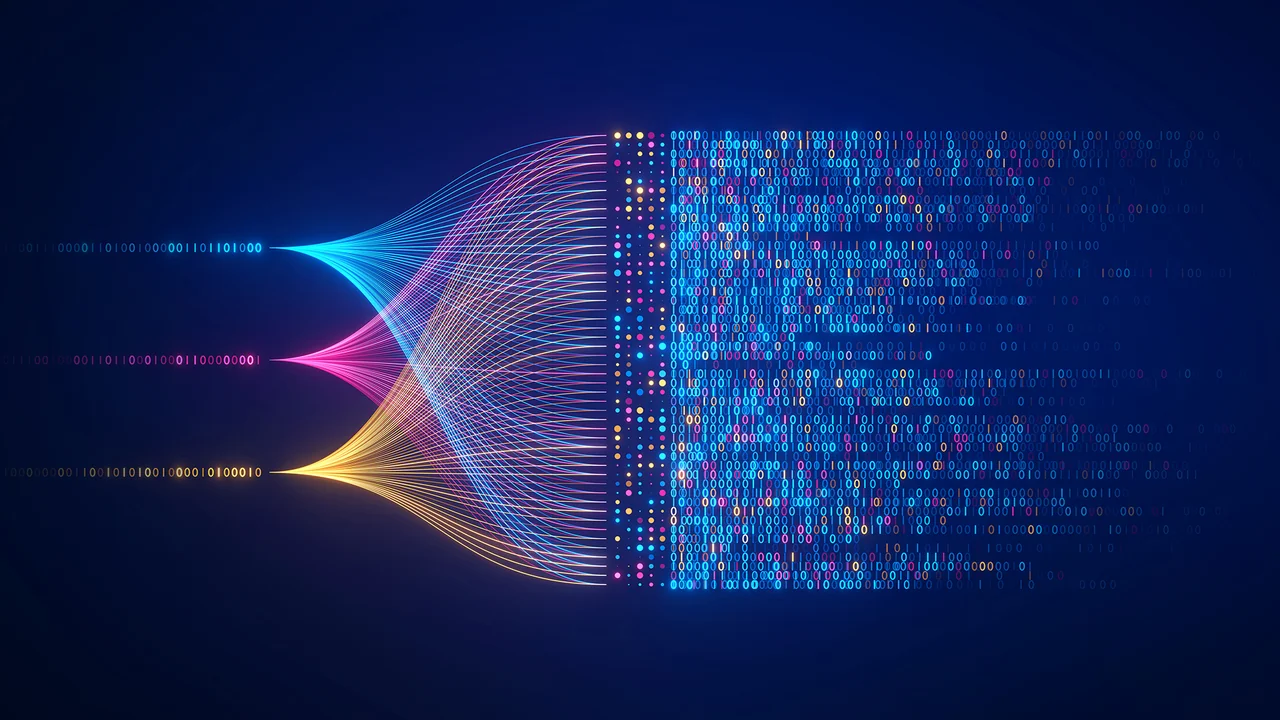
CTranslate2: Efficient Inference with Transformer Models on AMD GPUs
Optimizing Transformer models with CTranslate2 for efficient inference on AMD GPUs
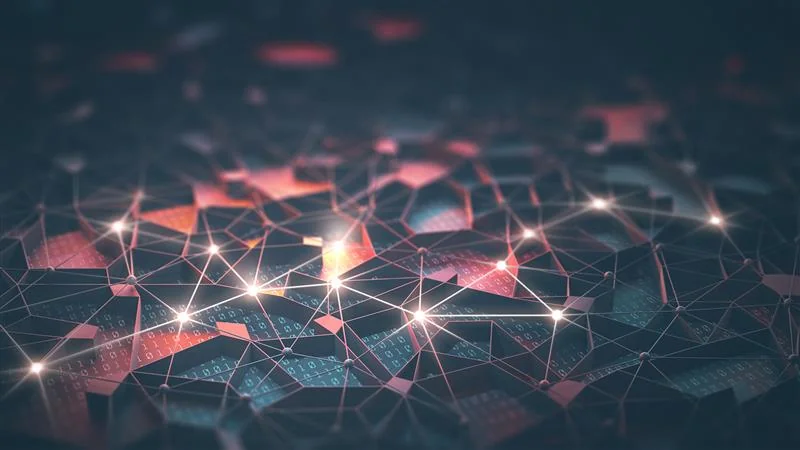
Inference with Llama 3.2 Vision LLMs on AMD GPUs Using ROCm
Meta's Llama 3.2 Vision models bring multimodal capabilities for vision-text tasks. This blog explores leveraging them on AMD GPUs with ROCm for efficient AI workflows.
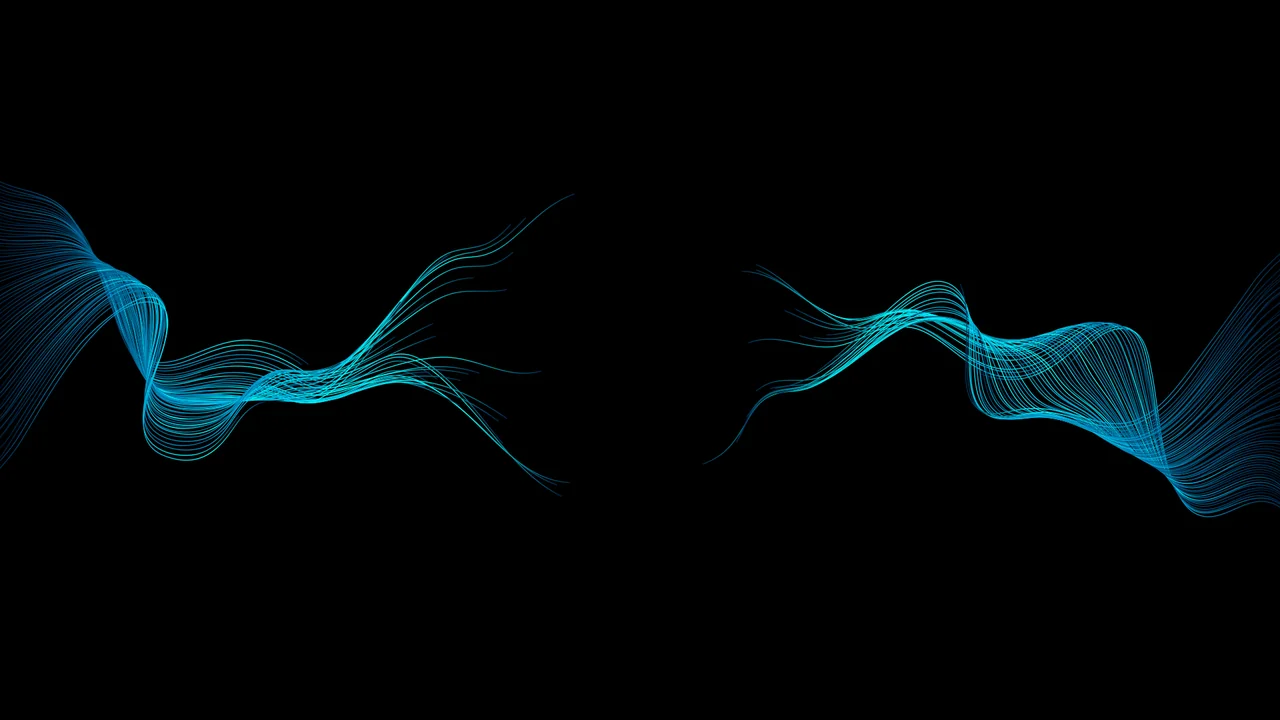
Speed Up Text Generation with Speculative Sampling on AMD GPUs
This blog will introduce you to assisted text generation using Speculative Sampling. We briefly explain the principles underlying Speculative Sampling and demonstrate its implementation on AMD GPUs using ROCm.
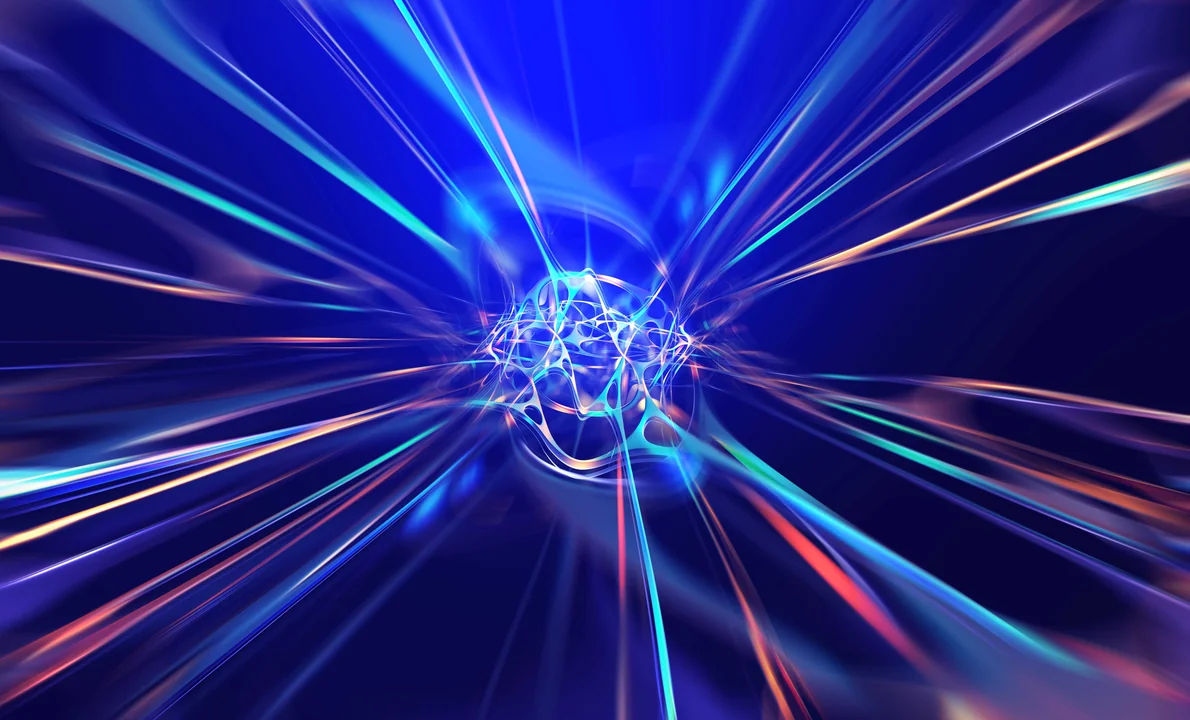
Multinode Fine-Tuning of Stable Diffusion XL on AMD GPUs with Hugging Face Accelerate and OCI's Kubernetes Engine (OKE)
This blog demonstrates how to set-up and fine-tune a Stable Diffusion XL (SDXL) model in a multinode Oracle Cloud Infrastructure’s (OCI) Kubernetes Engine (OKE) on a cluster of AMD GPUs using ROCm
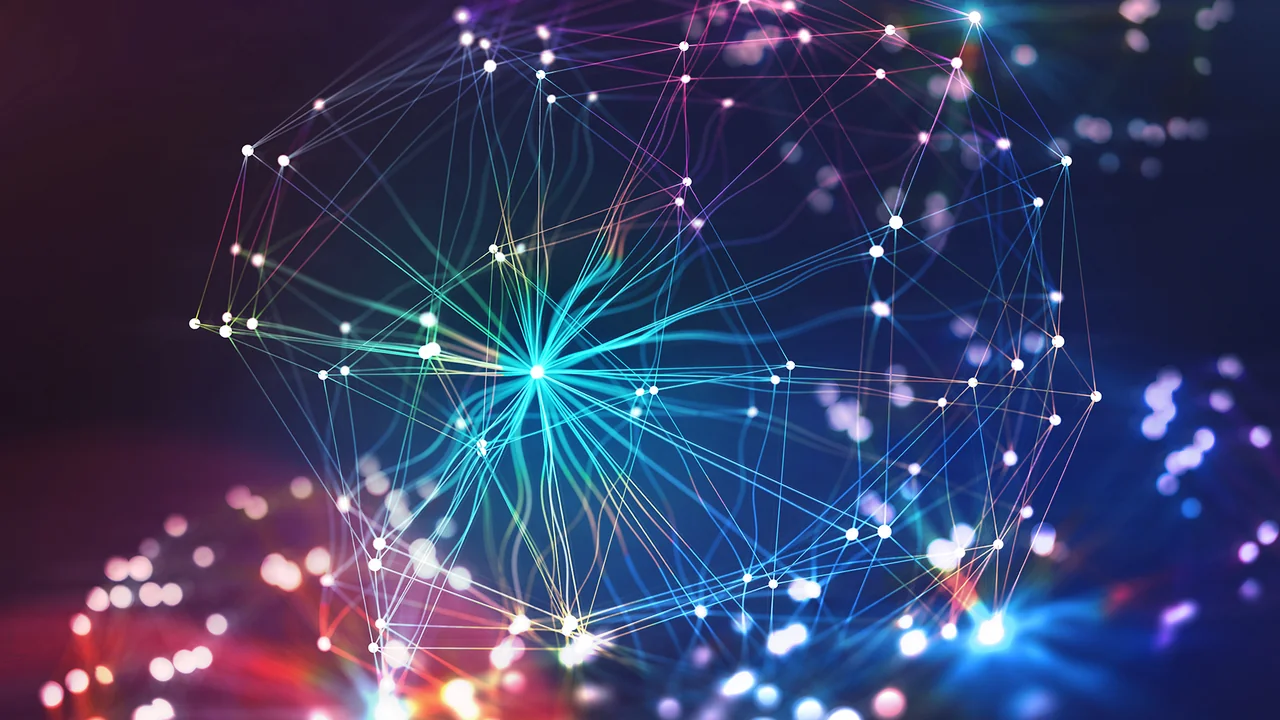
Enhancing vLLM Inference on AMD GPUs
In this blog, we’ll demonstrate the latest performance enhancements in vLLM inference on AMD Instinct accelerators using ROCm. In a nutshell, vLLM optimizes GPU memory utilization, allowing more efficient handling of large language models (LLMs) within existing hardware constraints, maximizing throughput and minimizing latency.
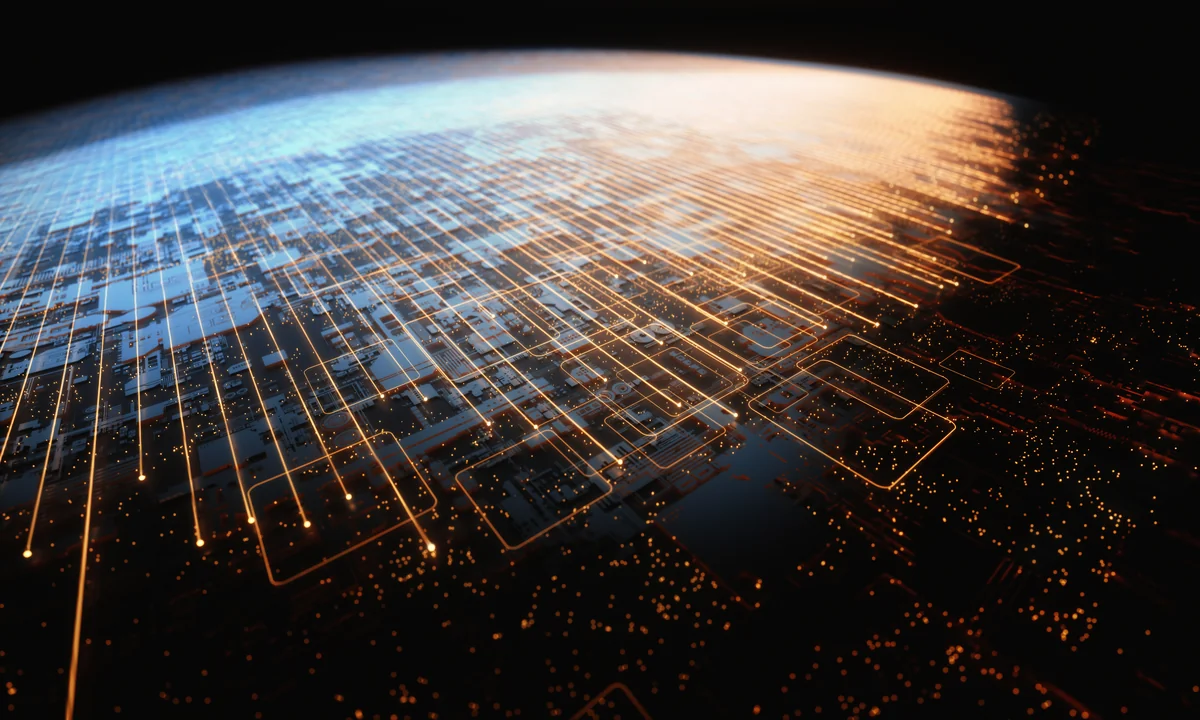
Supercharging JAX with Triton Kernels on AMD GPUs
In this blog post we guide you through developing a fused dropout activation kernel for matrices in Triton, calling the kernel from JAX, and benchmarking its performance.
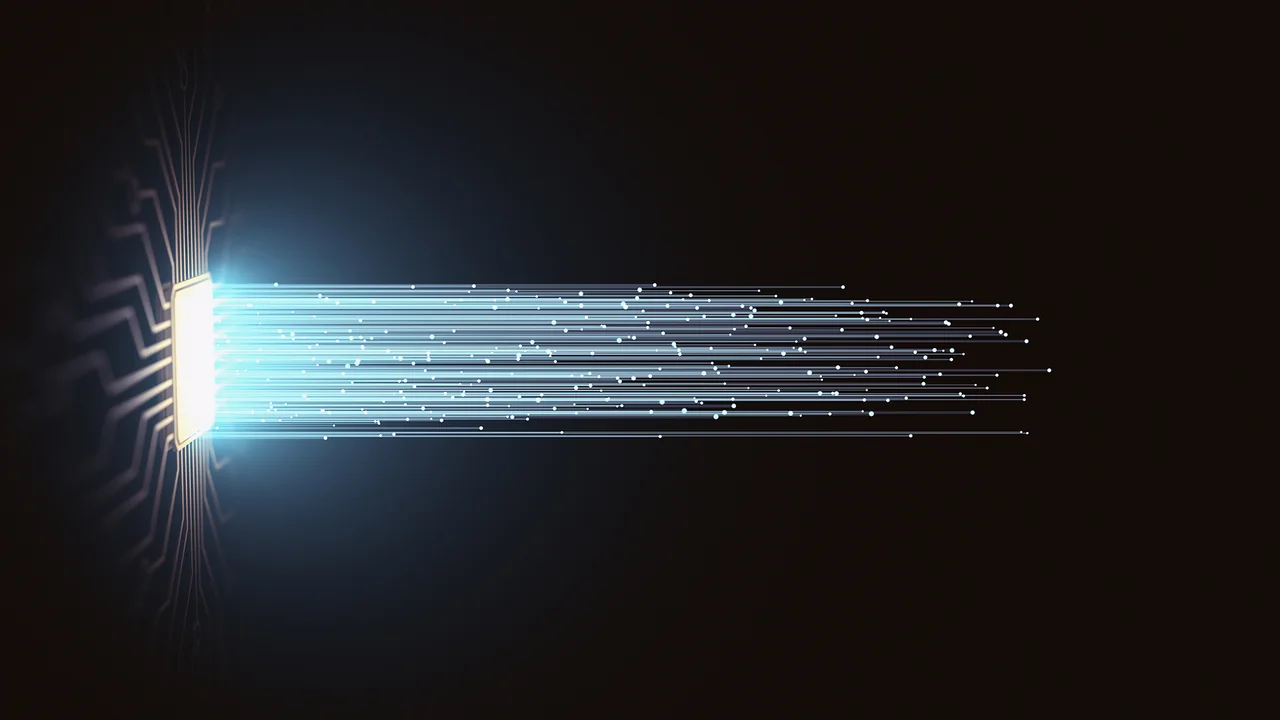
Leaner LLM Inference with INT8 Quantization on AMD GPUs using PyTorch
This blog demonstrates how to use AMD GPUs to implement and evaluate INT8 quantization, and the derived inference speed-up of Llama family and Mistral LLM models.
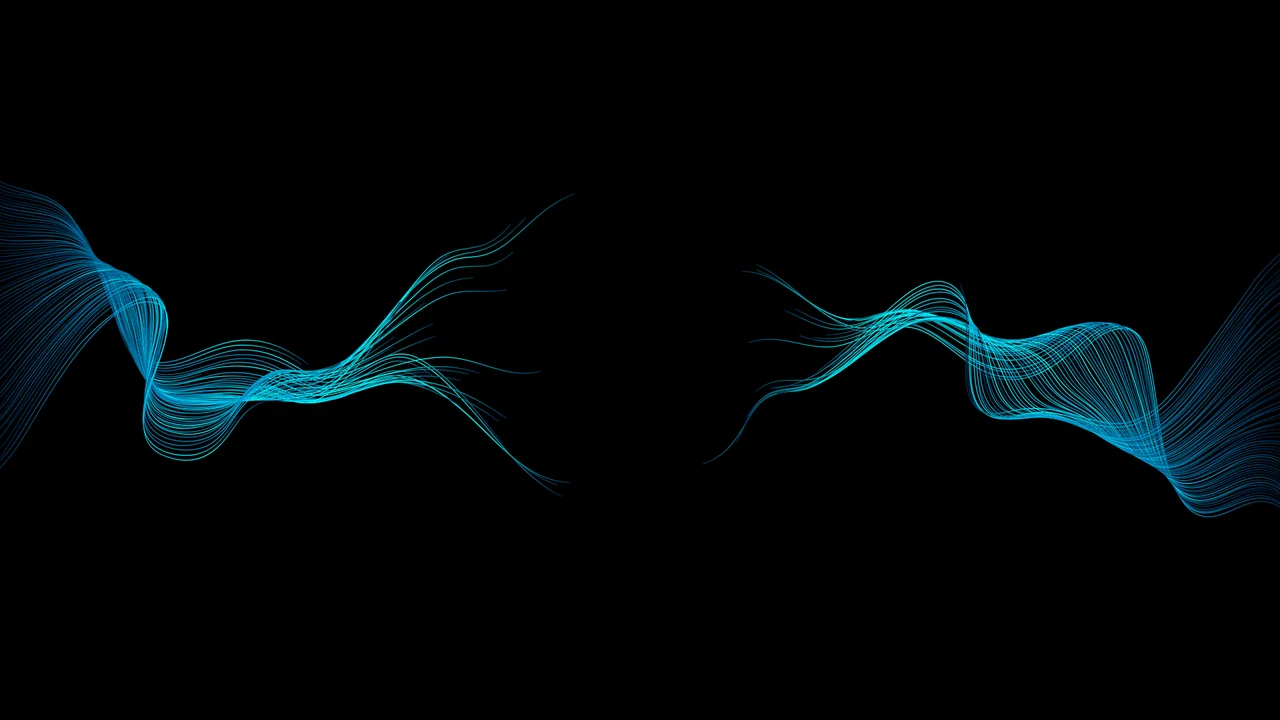
Fine-tuning Llama 3 with Axolotl using ROCm on AMD GPUs
This blog demonstrates how to fine-tune Llama 3 with Axolotl using ROCm on AMD GPUs, and how to evaluate the performance of your LLM before and after fine-tuning.
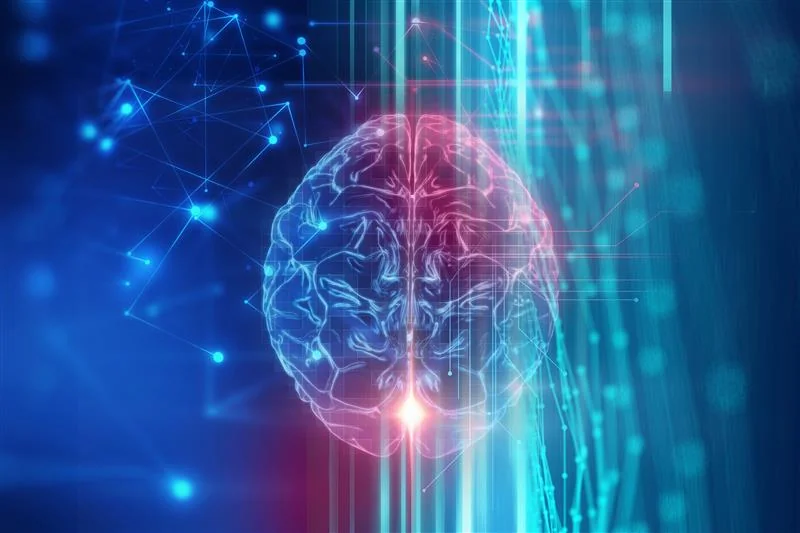
Inferencing and serving with vLLM on AMD GPUs
Inferencing and Serving with vLLM on AMD GPUs
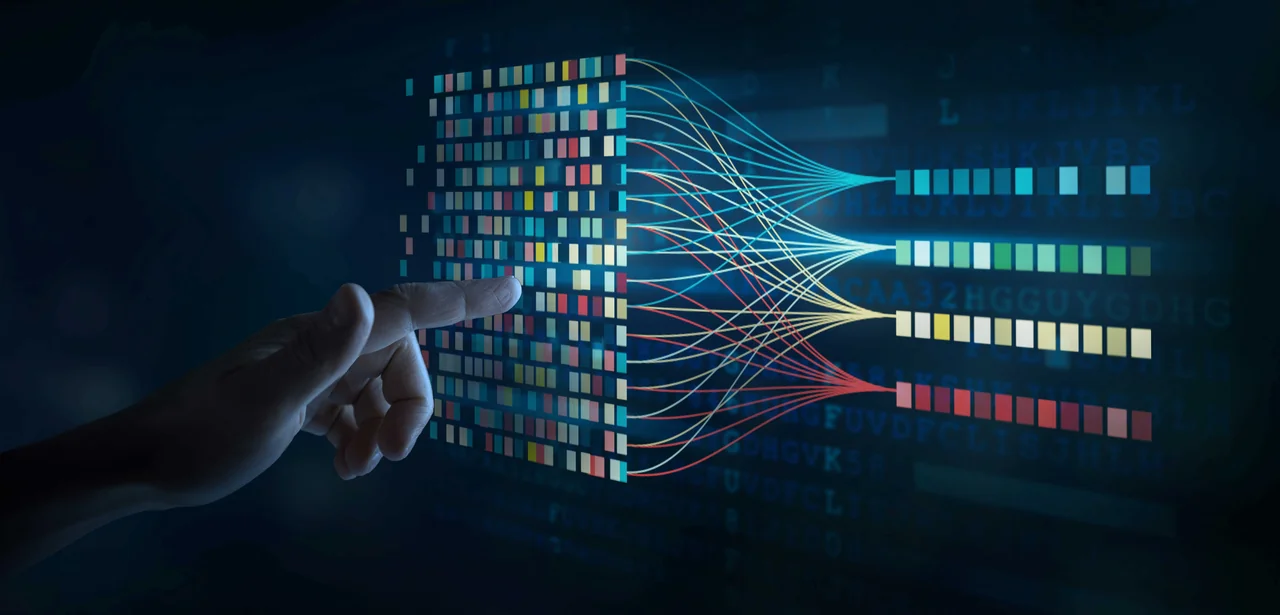
Optimize GPT Training: Enabling Mixed Precision Training in JAX using ROCm on AMD GPUs
Guide to modify our JAX-based nanoGPT model for mixed-precision training, optimizing speed and efficiency on AMD GPUs with ROCm.