Recent Posts#
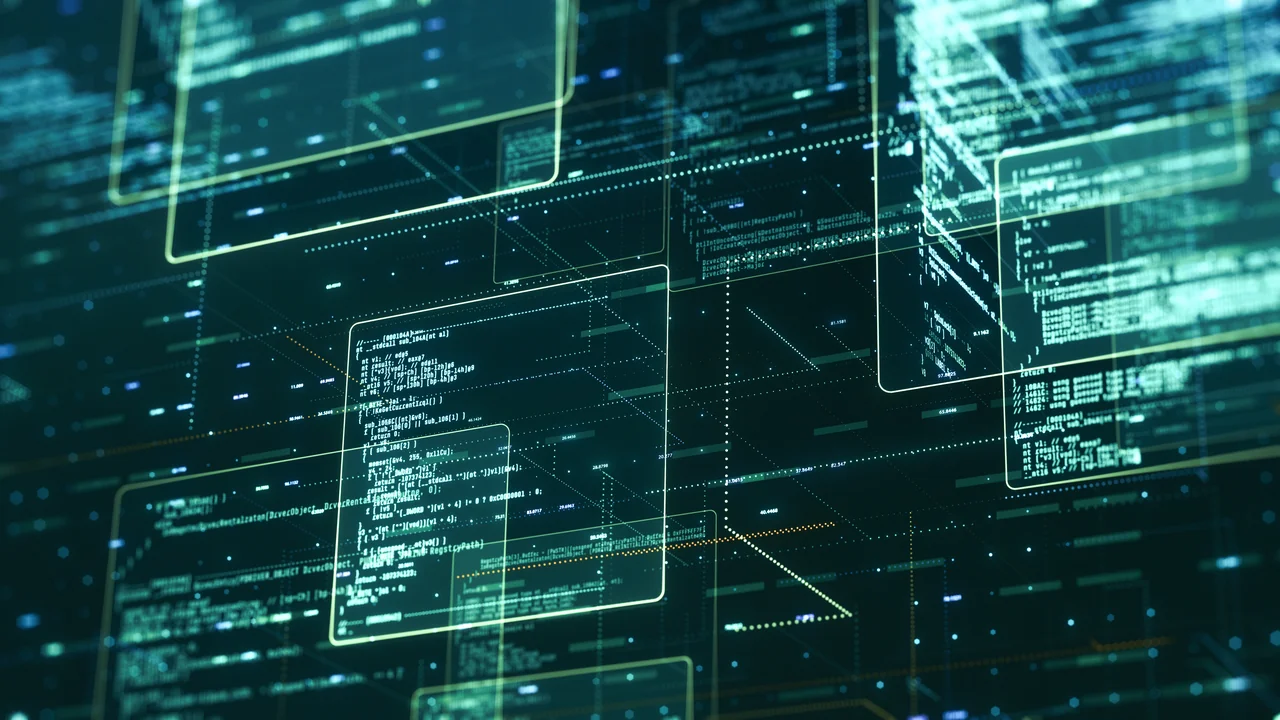
DataFrame Acceleration: hipDF and hipDF.pandas on AMD GPUs
This blog post demonstrates how hipDF significantly enhances and accelerates data manipulation, aggregation, and transformation tasks on AMD hardware using ROCm.
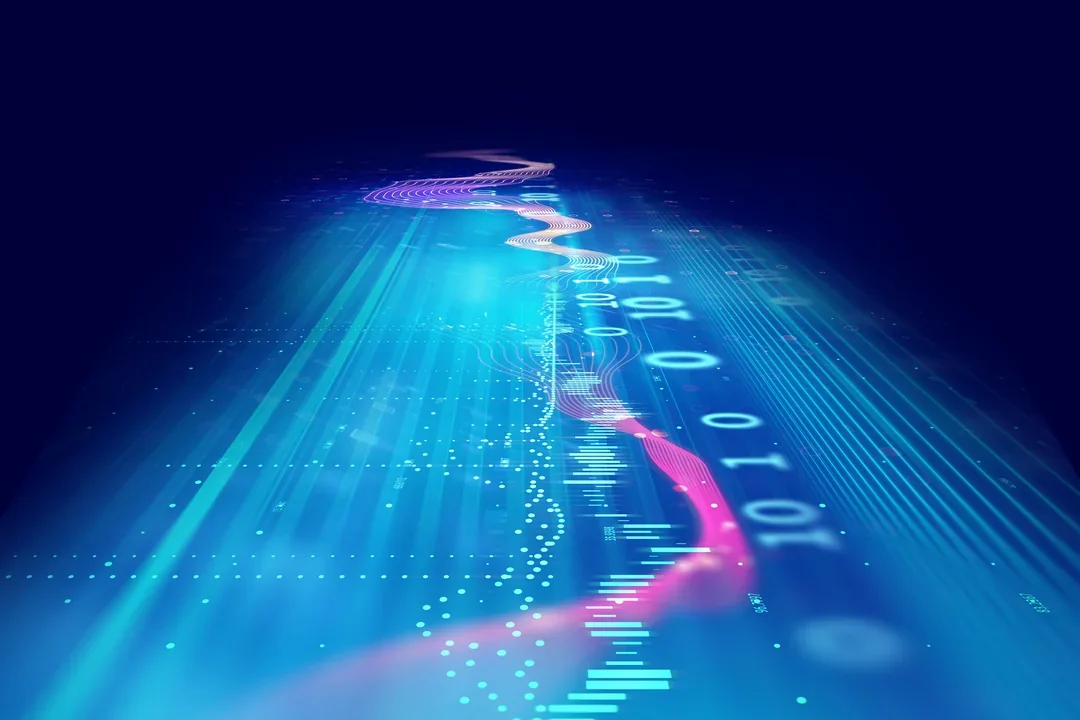
CuPy and hipDF on AMD: The Basics and Beyond
Learn how to deploy CuPy and hipDF on AMD GPUs. See their high-performance computing advantages, and use CuPy and hipDF in a detailed example of an investment portfolio allocation optimization using the Markowitz model.
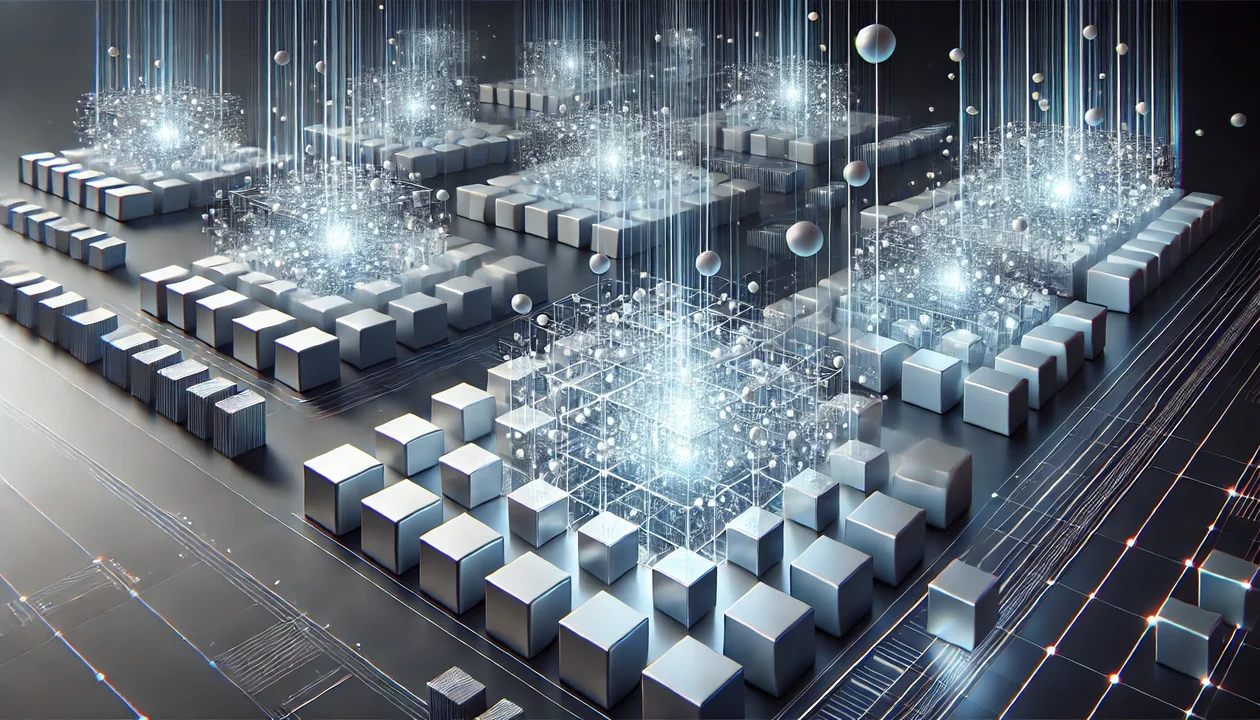
Unleash Full GPU Potential: Overlap Communication and Computation with Triton-Distributed
Unlock the full power of AMD GPUs—write portable, efficient kernels with Triton-Distributed, overlapping computation and communication with ease and flexibility
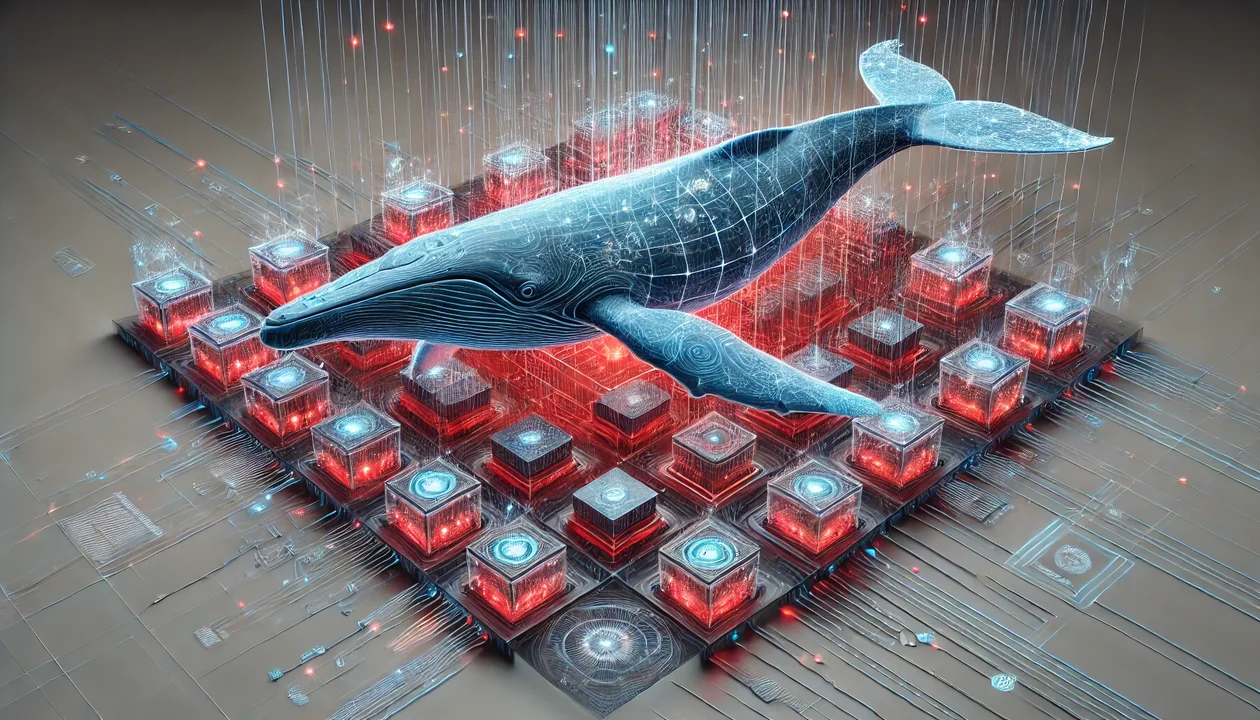
Optimizing DeepseekV3 Inference on SGLang Using ROCm Profiling Tools
Dive into kernel-level profiling of DeepseekV3 on SGLang—identify GPU bottlenecks and boost large language model performance using ROCm
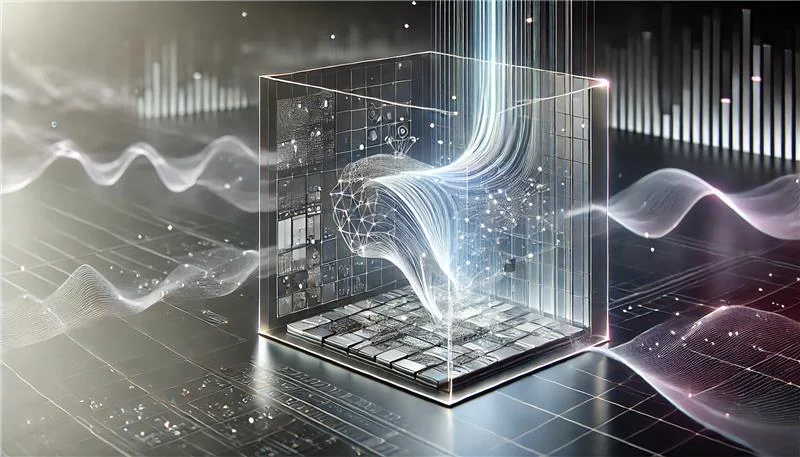
Power Up Qwen 3 with AMD Instinct: A Developer’s Day 0 Quickstart
Explore the power of Alibaba's QWEN3 models on AMD Instinct™ MI300X and MI325X GPUs - available from Day 0 with seamless SGLang and vLLM integration
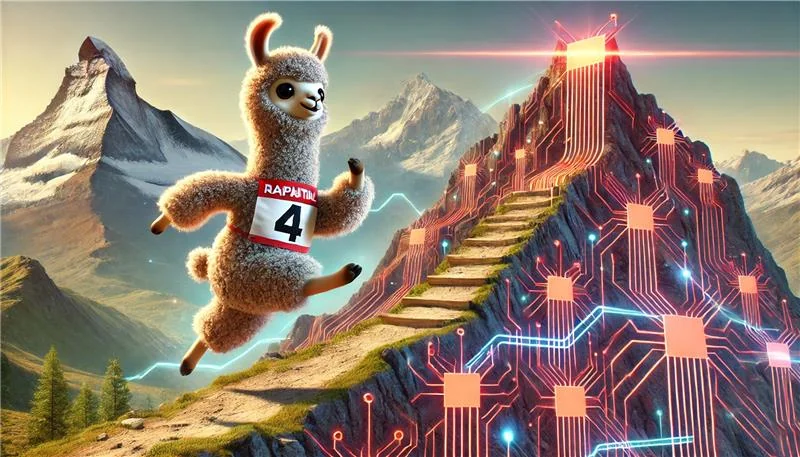
Boosting Llama 4 Inference Performance with AMD Instinct MI300X GPUs
Learn how to boost your Llama 4 inference performance on AMD MI300X GPUs using AITER-optimized kernels and advanced vLLM techniques
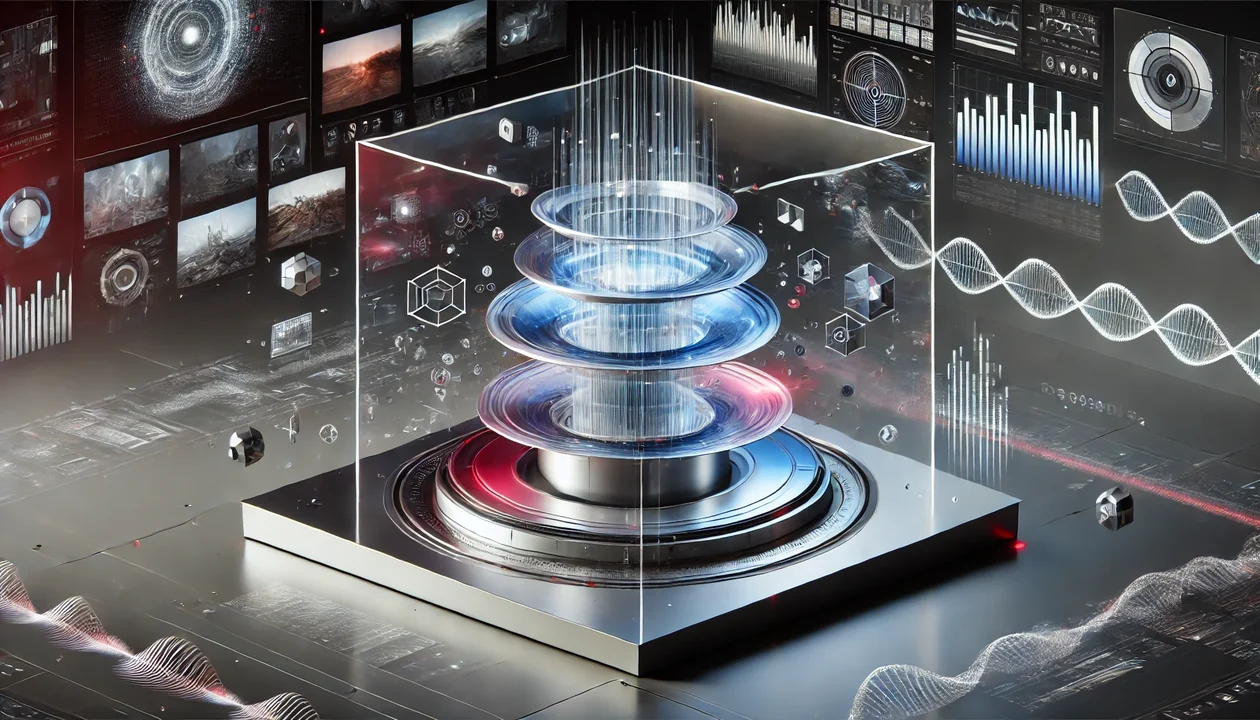
Beyond Text: Accelerating Multimodal AI Inference with Speculative Decoding on AMD Instinct™ MI300X GPUs
This blog shows you how to speedup your multimodal models with AMD’s open-source PyTorch tools for speculative decoding on MI300X GPUs
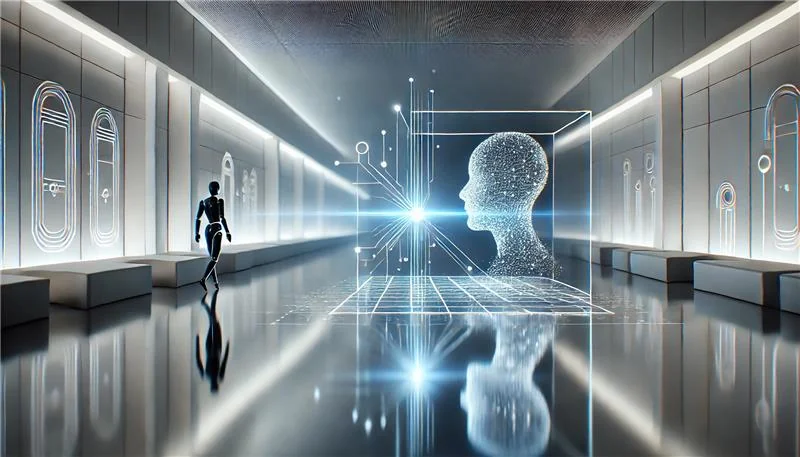
Reinforcement Learning from Human Feedback on AMD GPUs with verl and ROCm Integration
Deploy verl on AMD GPUs for fast, scalable RLHF training with ROCm optimization, Docker scripts, and impressive throughput-convergence results
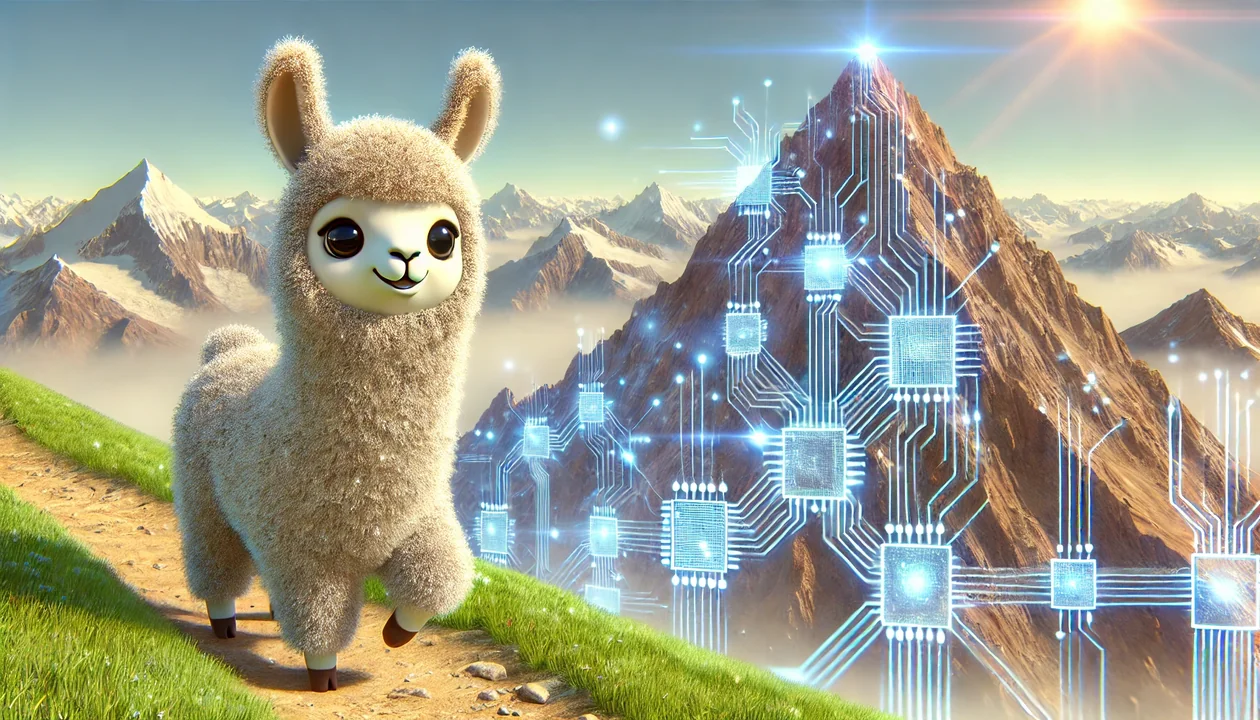
A Step-by-Step Guide On How To Deploy Llama Stack on AMD Instinct™ GPU
Learn how to use Meta’s Llama Stack with AMD ROCm and vLLM to scale inference, integrate APIs, and streamline production-ready AI workflows on AMD Instinct™ GPU
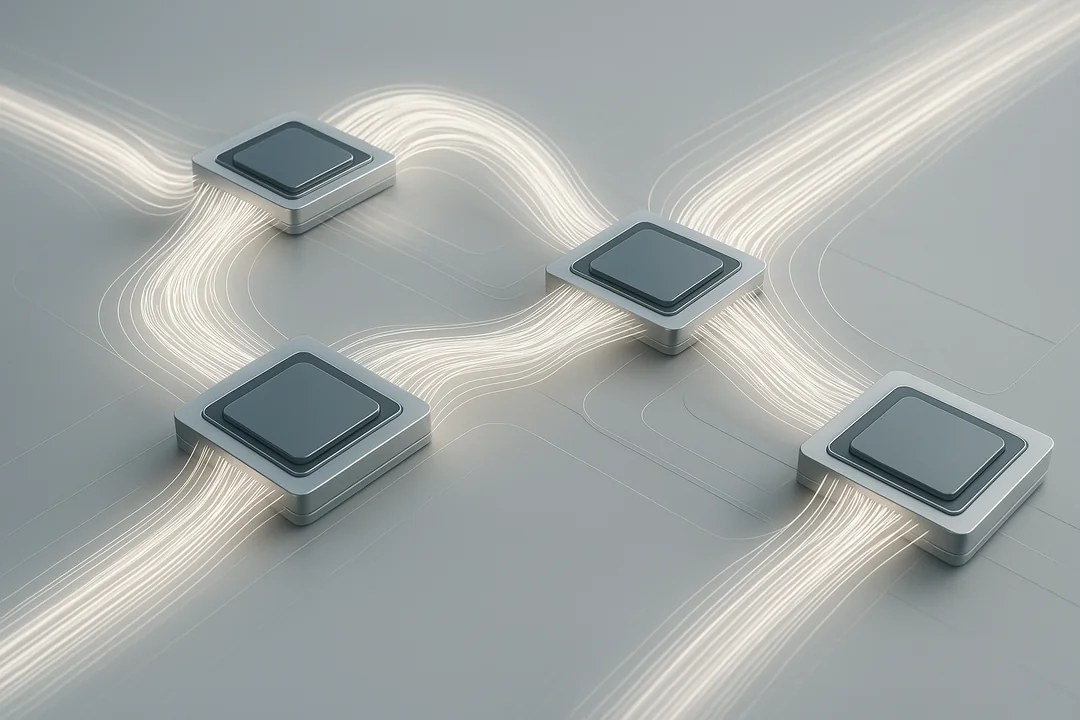
Hands-On with CK-Tile: Develop and Run Optimized GEMM on AMD GPUs
Build high-performance GEMM kernels using CK-Tile on AMD Instinct GPUs with vendor-optimized pipelines and policies for AI and HPC workloads
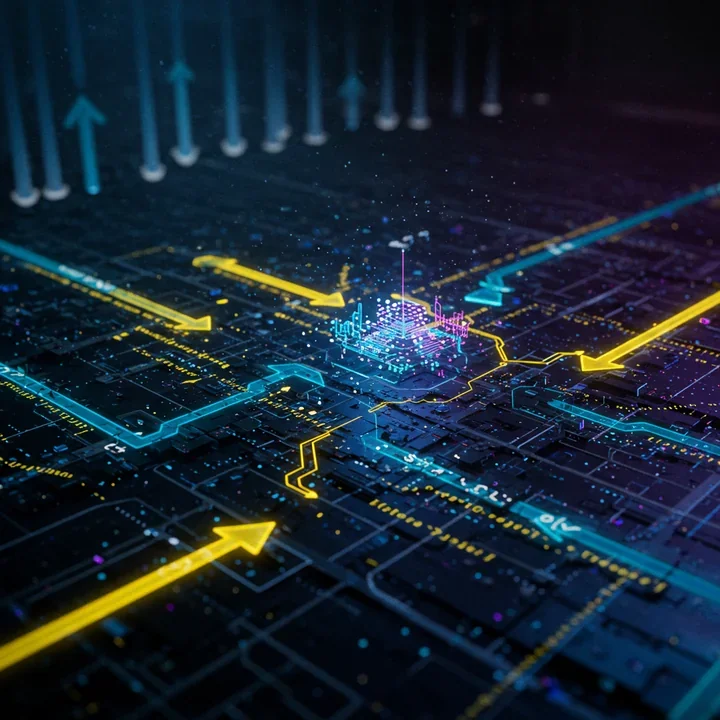
Installing ROCm from source with Spack
Install ROCm and PyTorch from source using Spack. Learn how to optimize builds, manage dependencies, and streamline your GPU software stacks.
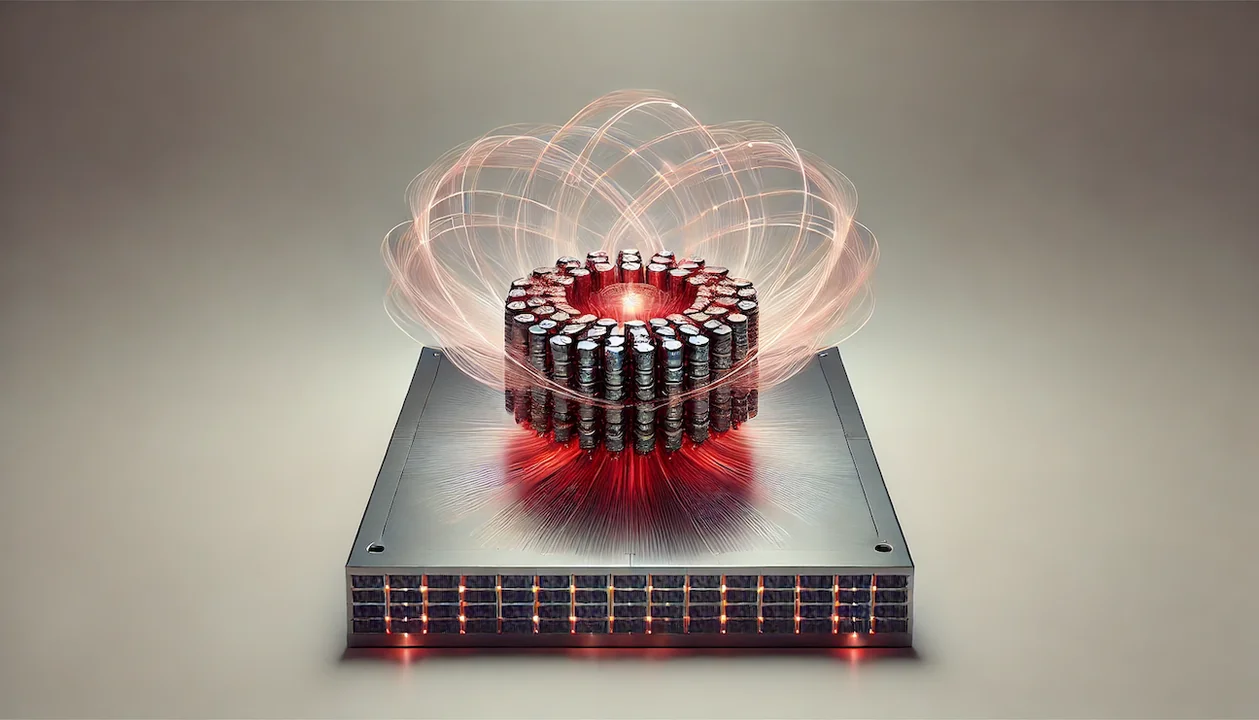
ROCm 6.4: Breaking Barriers in AI, HPC, and Modular GPU Software
Explore ROCm 6.4's key advancements: AI/HPC performance boosts, enhanced profiling tools, better Kubernetes support and modular drivers, accelerating AI and HPC workloads on AMD GPUs.